PhD Theses
Prosody has many functions in speech; e.g., cueing information structure (“Max bought a HOUSE.” vs. “MAX bought a house.”), sentence type (“Max bought a house?”), or communicative functions such as turn management (do I want to continue telling you about Max’s new house or am I done talking). This thesis investigates the prosody of yet another kind of communicative function, the expression of attitude (also called stance-taking, evaluation).
This thesis presents the analysis and evaluation of acoustic representations and models for conversational speech for two tasks: prosodic prominence classification and automatic speech recognition (ASR). Conversational speech poses unique challenges compared to read or prepared speech due to characteristics such as lively turn-taking, incomplete utterances, disfluencies, and high degree of pronunciation variation. Given these characteristics, both prosodic annotation tools and ASR systems trained on the typical benchmark datasets perform significantly worse on conversational speech. This thesis thus follows two aims, 1) to analyze acoustic representations for conversational speech using explainable machine learning (ML) methods, and 2) to improve the performance of prosodic prominence classification and ASR systems, as measured with standard performance measures. Our experiments on prosodic prominence classification revealed that the main acoustic cues for perceived prominence were the durational features. We introduce novel entropy-based prosodic features, which showed to encode necessary durational information along with information on pitch and loudness, leading to detection performances which aligned with inter-annotator agreements for the different prominence levels. These entropy-based prosodic representations were further used to examine their impact on utterance-level word error rates (WERs) of HMM- and transformer-based ASR systems. Our results reveal significant effects of durational and prosodic features on WER, but also how they interact with pronunciation variation and utterance-level complexity measures. Finally, we developed prominence detectors and prominence-aware ASR systems and explored how prosodic information is encoded through fine-tuning of self-supervised speech representations, indicating the feasibility of integrating prosodic information into ASR. Given that our experiments were based on data from conversational Austrian German, we had to deal with high variation stemming from dealing with a (low-resourced) regional variety of a (well-resourced) language in addition to the high variation between speakers and between different speaker pairs given the casual speaking style. Using clustering methods for shared discrete speech representations we demonstrated their effectiveness in differentiating language and variety aspects and capturing speaker differences across styles. The distances between quantized latent speech representations showed to meaningfully capture fine-grained differences between speakers when producing different speaking styles. Overall, this thesis provides insights into the complexities of conversational speech and demonstrates how the analysis and evaluation of acoustic representations and models deepen our understanding of conversational speech. The findings have implications for various applications such as human-machine interaction, conversation transcription and hearing aid technology.
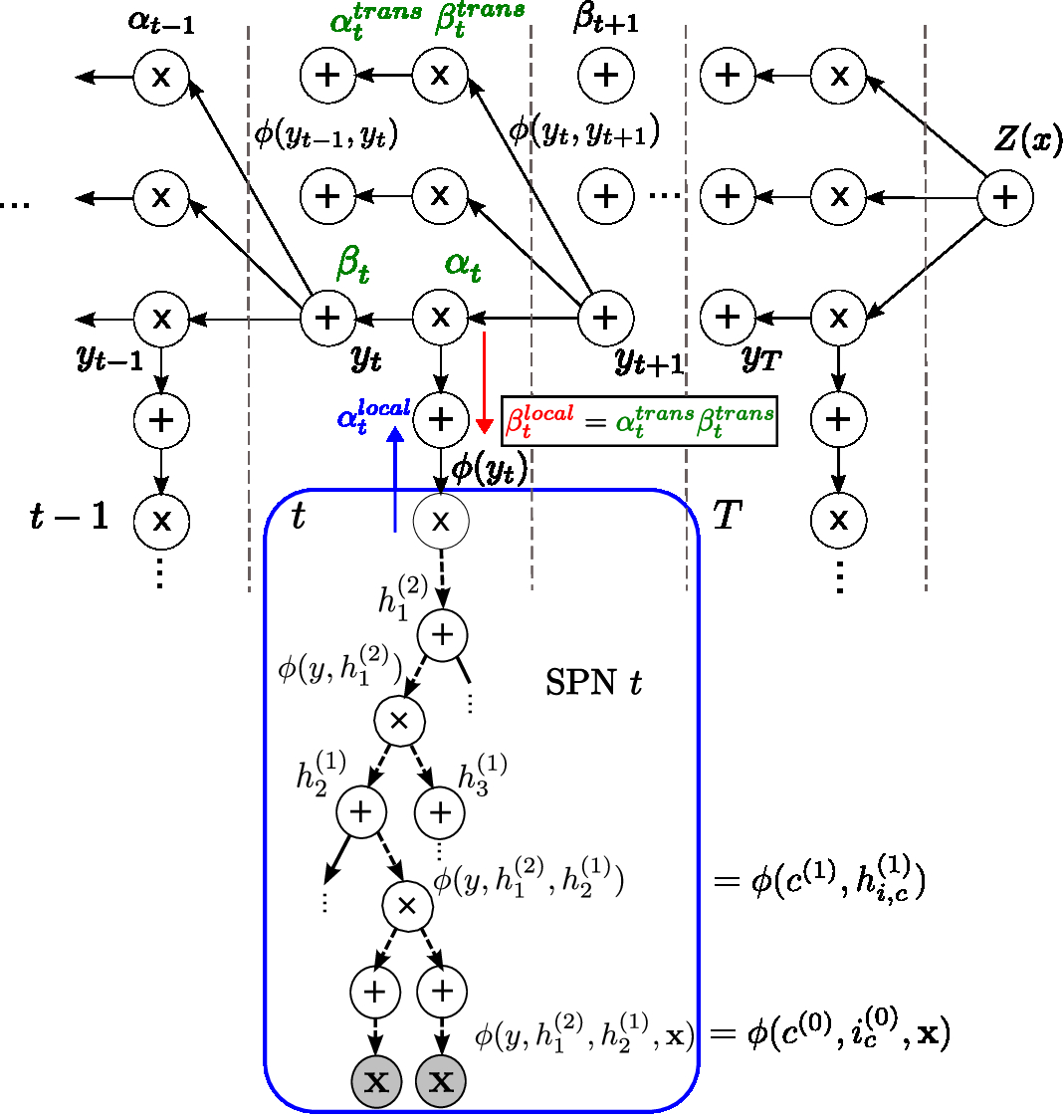
Linear-chain conditional random fields (LC-CRFs) have been successfully applied in many structured prediction tasks. LC-CRFs can be extended by different types of deep models.
Edge absorbers are known for their high effectiveness in absorbing low-frequency sound energy. Particular attention must be paid to low-frequency sound energy and especially low-frequency reverberation when planning and/or renovating communication rooms, as these have a sensitive effect on speech intelligibility due to masking effects. Here, edge absorbers, commonly known as bass traps, can be used as a subtle and relatively inexpensive acoustic treatment. Although the influence of edge absorbers on the sound field and its decay behaviour has been extensively proven empirically, no suitable modelling of the edge absorber exists to date. For this reason, edge absorbers are hardly ever used in room acoustic simulations.
When speaking spontaneously, we often reduce articulatory precision, put less effort into producing flawless sentences, or utter disfluent structures. As humans, we are usually still able to decode (understand) such imperfect utterances. One reason for that is that we have been learning to deal with spoken language during our lifetime which provides us with powerful speech processing models. An automatic speech recognition (ASR) system, in contrast, is much more limited to the (finite amount of) data it was trained on. Another reason is that humans can fall back on context and the history of a conversation which helps them to evaluate the plausibility of (sequences of) words in given surroundings and thus untangle probable disambiguations. ASR systems achieve accuracies close to human performance for read speech. When it comes to recognising spontaneous speech, however, systems perform much worse. The last notable advancement in ASR has been the introduction of transformer-based systems, which deliver impressive results on established conversational speech databases. These systems were trained on huge amounts of data and make use of a wider context; therefore, one could expect that they should be able to solve the last remaining challenges on conversational speech recognition. When it comes to recognising spontaneous, unscripted conversations, however, they still yield surprisingly high word error rates. This thesis analyses the similarities and differences between transcription errors made by ASR systems and those made by human transcribers. It aims at finding where we can still learn from human performance and linguistic knowledge and encourages further research on speech from unscripted, spontaneous conversations.
Upon asking what kind of problems hearing aid users have when listening to music, most of the answers will be that some instruments are too loud, some too soft, or that it is all one big mush. The field of musical scene analysis (MSA) investigates the human perceptual ability to organize complex musical structures, such as the sound mixtures of an orchestra, into meaningful lines or streams from its individual instruments or sections. Many studies have already been performed on various MSA-tasks for humans as it bears the key to better understand music perception and help improve the enjoyment of music in hearing impaired people. For example, Siedenburg et al. (2020, 2021) demonstrated the effect of instrumentation on the ability to track instruments in artificial and natural musical mixtures. Bürgel et al. (2021) showed that lead vocals in pop music especially attract attention of the listener. Furthermore, Hake et al. (2023) presented results of MSA tests that differed depending on the participant’s level of hearing loss. However, there are still many open questions. One key question concerns the acoustical features underpinning MSA in natural music the human ear and brain use to selectively filter out single instruments/voices from sound mixtures. The goal of my PhD research is to create a signal-based model that accounts for response behavior of human listeners when asked if a sound from a target instrument can be heard in a musical mixture. I seek to analyze methods of the auditory apparatus to process music and study the ways in which they are hindered by sensorineural hearing loss. As a starting point I will be using existing models for speech perception and audio quality that use features such as linear and non-linear filterbanks, modulation filterbanks, and envelope extraction that simulate the auditory processing. Drawing from previous experiments, model performance is assessed by evaluating fit to human performance. The resulting model might then be used to test algorithms to improve selective hearing in music and provide a detailed picture on how humans perceive music.
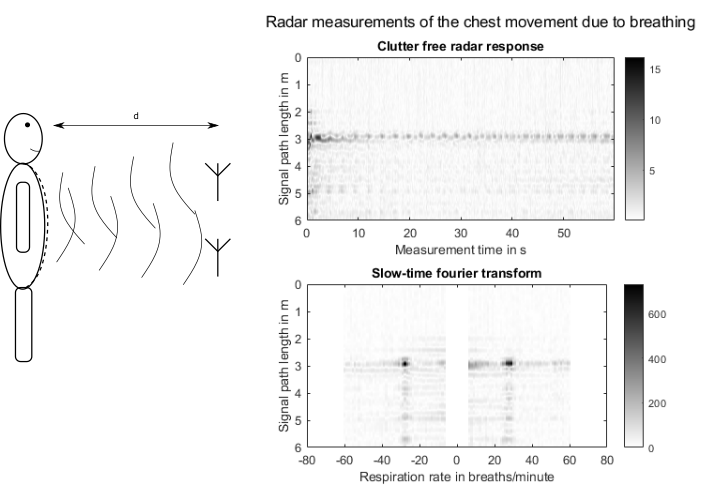
Approximatley 40 tragic deaths of small children locked in vehicles occur in the US each year due to the extreme heat or cold inside the parked vehicle. If the vehicle is able to detect the presence of a child (or any other life such e.g. a pet) it can either alert the owner or adjust the climate control in order to avoid these tragic accidents.
Voice plays a fundamental role in human communication, not only serving a functional purpose but also shaping personal identity and social interaction. Voice disorders, such as dysphonia or conditions resulting from laryngeal cancer, can severely impact the ability to communicate, often leading to social isolation and psychological burdens. In cases requiring a laryngectomy, patients rely on electro-larynx (EL) devices, which generate unnatural, robotic speech that hinders effective interaction. This research explores the potential of voice conversion (VC) models to enhance speech quality for individuals with pathological voices, bridging the gap between assistive technology and natural communication. While state-of-the-art VC models exist, few are optimized for medical applications, particularly in real-time streaming scenarios. A key focus of this work is developing low-latency, high-quality VC models tailored for pathological speech, including EL voice conversion. By improving the efficiency and adaptability of VC systems, this research aims to push the boundaries of speech synthesis and enable real-world applications that enhance communication for individuals with voice disorders.
Finished Theses
- 2023: Interpretable Fault Prediction for CERN Energy Frontier Colliders — Christoph Obermair
- 2022: Narrowband positioning exploiting massive cooperation and mapping — Lukas Wielandner
- 2022: Robust Positioning in Ultra-Wideband Off-Body Channels — Thomas Wilding
- 2022: Deep Learning for Resource-Constrained Radar Systems — Johanna Rock
- 2022: Robust Lung Sound and Acoustic Scene Classification — Truc Nguyen
- 2021: Towards the Evolution of Neural Acoustic Beamformers — Lukas Pfeifenberger
- 2021: Signal Processing for Localization and Environment Mapping — Michael Rath
- 2020: Evaluating the decay of sound — Jamilla Balint
- 2020: Cognitive MIMO Radar for RFID Localization — Stefan Grebien
- 2019: Speech Enhancement Using Deep Neural Beamformers — Matthias Zöhrer
- 2019: Contributions to Single-Channel Speech Enhancement with a Focus on the Spectral Phase — Johannes Stahl
- 2017: Localization, Characterization, and Tracking of Harmonic Sources: With Applications to Speech Signal Processing — Hannes Pessentheiner
- 2015: The Bionic Electro-Larynx Speech System - Challenges, Investigations, and Solutions — Anna Katharina Fuchs
- 2014: Diplophonic Voice: Definitions, models, and detection — Philipp Aichinger
- 2013: Kernel PCA and Pre-Image Iterations for Speech Enhancemen — Christina Leitner
- 2012: Probabilistic Model-Based Multiple Pitch Tracking of Speech — Michael Wohlmayr
- 2011: Auditory Inspired Methods for Multiple Speaker Localization and Tracking Using a Circular Microphone Array — Tania Habib
- 2010: Source-Filter Model Based Single Channel Speech Separation — Michael Stark
- 2010: Phonetic Similarity Matching of Non-Literal Transcripts in Automatic Speech Recognition — Stefan Petrik
- 2009: Speech Enhancement for Disordered and Substitution Voices — Martin Hagmüller
- 2009: Speech Watermarking and Air Traffic Control — ~Konrad Hofbauer
- 2007: Variable Delay Speech Communication over Packet-Switched Networks — ~Muhammad Sarwar Ehsan
- 2007: Semantic Similarity in Automatic Speech Recognition for Meetings — Michael Pucher
- 2007: Wavelet Analysis For Robust Speech Processing and Applications — Van Tuan Pham
- 2006: Quality Aspects of Packet-Based Interactive Speech Communication — Florian Hammer
- 2005: Sparse Pulsed Auditory Representations For Speech and Audio Coding — Christian Feldbauer
- 2003: Improving automatic speech recognition for pluricentric languages exemplified on varieties of German — ~Micha Baum
- : UWB Channel Fading Statistics and Transmitted Reference Communication — N.N.
- : Signal Processing in Phase-Domain All-Digital Phase-Locked Loops — N.N.
- : Signal Processing for Ultra Wideband Transceivers — N.N.
- : Signal Processing for Burst‐Mode RF Transmitter Architectures — Katharina Hausmair
- : Reliable and Robust Localization and Positioning — Alexander Venus
- : Probabilistic Methods for Resource Efficiency in Machine Learning — Wolfgang Roth
- : Position Aware RFID Systems — Daniel Arnitz
- : Understanding the Behavior of Belief Propagation — Christian Knoll
- : Nonlinear System Identification for Mixed Signal Processing — N.N.
- : Multipath Tracking and Prediction for Multiple-Input Multiple-Output Wireless Channels — Daniel Arnitz
- : Multipath-Assisted Indoor Positioning — Paul Meissner
- : Modeling, Identification, and Compensation of Channel Mismatch Errors in Time-Interleaved Analog-to-Digital Converters — Christian Vogel
- : Modeling and Mitigation of Narrowband Interference for Non-Coherent UWB Systems — ~Yohannes Alemseged Demessie
- : Measurement Methods for Estimating the Error Vector Magnitude in OFDM Transceivers — Karl Freiberger
- : Maximum Margin Bayesian Networks — Sebastian Tschiatschek
- : Low Complexity Ultra-wideband (UWB) Communication Systems in Presence of Multiple-Access Interference — ~Jimmy Wono Tampubolon Baringbing
- : Low-Complexity Localization using Standard-Compliant UWB Signals — N.N.
- : Low Complexity Correction Structures for Time-Varying Systems — Michael Soudan
- : Information Theory for Signal Processing — Bernhard Geiger
- : Indoor localization using RF channel information — Josef Kulmer
- : Improving Efficiency and Generalization in Deep Learning Models for Industrial Applications — Alex Fuchs
- : Foundations of Sum-Product Networks for Probabilistic Modeling — Robert Peharz
- : Efficient Floating-Point Implementation of Speech Processing Algorithms on Reconfigurable Hardware — Thang Huynh Viet
- : Distributed Sparse Bayesian Regression in Wireless Sensor Networks — Thomas Buchgraber
- : Digital Enhancement and Multirate Processing Methods for Nonlinear Mixed Signal Systems — N.N.
- : Complex Baseband Modeling and Digital Predistortion for Wideband RF Power Amplifiers — ~Peter Singerl
- : Cognitive Indoor Positioning and Tracking using Multipath Channel Information — Erik Leitinger
- : Behavioral Modeling and Digital Predistortion of Radio Frequency Power Amplifiers — Harald Enzinger
- : Sum-Product Networks for Complex Modelling Scenarios — Martin Trapp
- : Adaptive Digital Predistortion of Nonlinear Systems — ~Lee Gan
- : Adaptive Calibration of Frequency Response MIsmatches in Time-Interleaved Analog-to-Digital Converters — Shahzad Saleem
- : A Holistic Approach to Multi-channel Lung Sound Classification — Elmar Messner