Deep Learning for Resource-Constrained Radar Systems
- Status
- Finished
- Student
- Johanna Rock
- Mentor
- Franz Pernkopf
- Research Areas
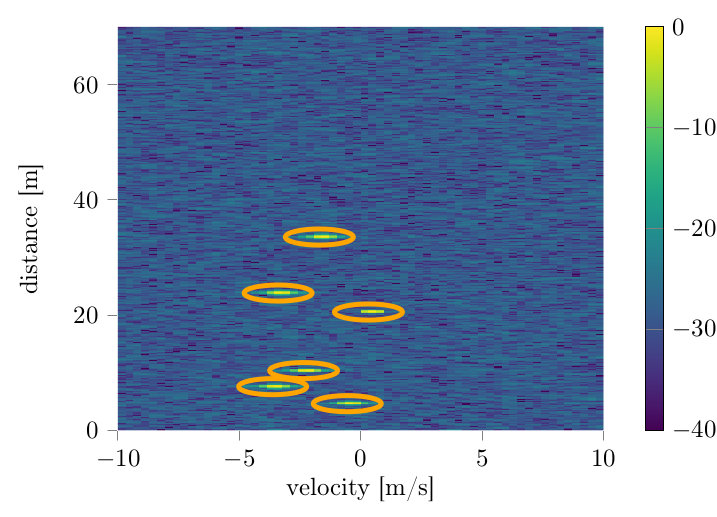
Radar sensors are crucial for environment perception of advanced driver assistance systems and autonomous driving. With a rising number of deployed radar sensors and due to missing radar transmit protocols, an increasing number of radar sensors is expected to send with overlapping frequencies. If multiple radar sensors send with overlapping frequencies at the same time, this leads to mutual interference, and subsequently, to a decreased detection sensitivity in the radar signal. Therefore, mutual interference must be dealt with to ensure the safe functionality of self-driving technology. Signal processing methods for radar technology are required to run directly on specialized sensor hardware to ensure real-time capability and to provide high-quality data for higher-level algorithms. Typically, this specialized hardware has strict resource constraints, i.e. low memory and computational capabilities, introducing additional constraints on interference mitigation algorithms.
In this thesis, we propose a convolutional neural network-based approach for interference mitigation and denoising of automotive radar signals. We contrast different data representations, neural architectures, and configurations with a focus on interference mitigation quality and resource efficiency. In early experiments, we used simulated object signals and interferences to simplify data acquisition and gain access to input-output pairs for training Neural Networks in a supervised learning paradigm. The object signals were later replaced by real-world measurements from inner-city traffic scenarios for a more realistic experimental setup. We analyze similarities and dissimilarities in simulated and real-sensor interferences and additionally present exemplary results for interference mitigation on measurements with real-sensor interference. Based on our analysis and experimental results, we argue for the applicability of our approach to real-world radar signals. Furthermore, we select a well-performing small architecture based on its memory footprint and interference mitigation performance and further compress this model using quantization. In our experiments, we use quantization aware training with the straight-through gradient estimator to decrease the bit width, i.e. the number of bits required to represent weights and activations, without impairing performance. We achieve a memory reduction of around 80% compared to the real-valued baseline by quantizing weights and activations with hetero- geneous bit widths, i.e. a different bit width in each layer. Due to practical reasons, however, we recommend the use of 8 bits for weights and activations in all layers, which results in a memory reduction of around 75% compared to the real-valued baseline, i.e. a model that requires only 0.2 megabytes of memory. The real-valued baseline and the 8-bit quantized model, outperform state-of-the-art signal processing-based interference mitigation methods on both simulated and measured radar signals.