Robust Lung Sound and Acoustic Scene Classification
- Status
- Finished
- Student
- Truc Nguyen
- Mentor
- Franz Pernkopf
- Research Areas
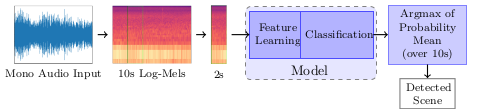
Auscultation with a stethoscope enables us to recognize pathological changes of the lung. It is a fast and inexpensive diagnosis methods. However, it has several disadvantages: subjectiveness, i.e. the lung sound evaluation depends on the experience of physicians, can not provide continuous monitoring and a trained expert is required. Furthermore, the characteristics of the lung sounds are in the low frequency range, where the human hearing has limited sensitivity and is susceptible to noise artifacts. Exploiting the advances in digital recording devices, signal processing and machine learning, computational methods for the analysis of lung sounds have been a successful and effective approach. Computational lung sound analysis is beneficial for computer-supported diagnosis, digital storage and monitoring in critical care.
Beside computational lung sound analysis, the recognition of acoustic contextual information is important in various applications. The motivation for recent research on acoustic scene classification is in designing a system that enables to automatically capture and exploit the specific properties of a given audio scene. These algorithms are embedded in commercial smart devices with microphones. However, in real environments, acoustic scenes are unstructured and often unpredictable in its occurrence causing many challenges for acoustic scene classification. To facilitate a more objective assessment of the lung sounds for diagnosis of pulmonary diseases/conditions and of environmental acoustic scenes, this thesis explores machine learning methods for sound classification. The objective is to address challenges of a large public lung sound dataset ICBHI 2017, our multi-channel lung sound dataset and acoustic scene data from DCASE challenges in order to achieve competitive performance compared to the state-of-the-art systems.
In particular, different topics of machine learning are explored as follows: (i) Deep neural networks with single input, multi-input and resource-efficient architectures are proposed to automatically discover the learned representations for both classification of lung sounds and acoustic scenes. (ii) We exploit different approaches of transfer learning to make use of knowledge from a large dataset for our proposed lung sound classification systems. In particular, we present a multi-input deep neural network built from a pre-trained single-input model. This allows to simultaneously assess information of adventitious lung sounds in a respiratory cycle as well as its phases. Beside popular transfer learning techniques, we also exploit co-tuning and stochastic normalization in order to transfer more knowledge from pre-trained models. (iii) Furthermore, different ensemble techniques have been used. A novel snapshot ensemble is applied to enhance the robustness of lung sound classification while keeping the drawback of expensive training of ensembles moderate. Classical ensemble techniques have been exploited and led to successes in acoustic scene classification, in particular, we won the DCASE 2018 challenge task of acoustic scene classification for mismatched recording devices. (iv) In addition, data augmentation, which accounts for data limitations and benefits deep learning models, has been used in all our works for performance enhancement.
All proposed systems have been experimentally evaluated on lung sound and acoustic scene datasets. We demonstrate the respective performance using metrics such as accuracy and F-score.