Probabilistic Graphical Models
- Research Areas
- Contact
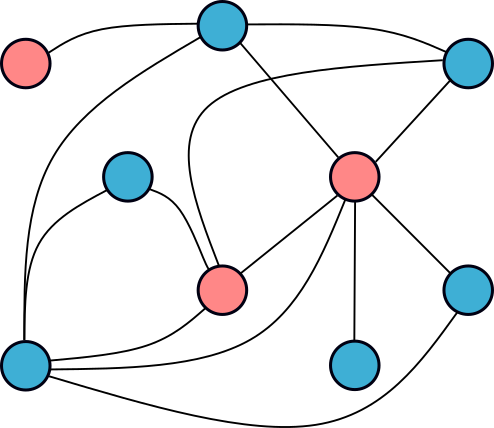
Probabilistic graphical models unite probability and graph theory and allow to formalize static and dynamic, as well as linear and nonlinear systems and processes. Many well-known statistical models (e.g. mixture models, factor analysis, hidden Markov models, Kalman filters, Bayesian networks, Boltzmann machines, and the Ising model) have a natural representation as graphical models. The framework of graphical models is highly flexible in representing structure and provides specialized techniques for inference and learning. This makes graphical models relevant for a wide range of research areas.
Learning: There are two basic approaches for learning graphical models in the scientific community: generative and discriminative learning. Unfortunately, generative learning does not always provide good results. Discriminative learning is known to be more accurate for classification. In contrast to discriminative models (e.g. neural networks, support vector machines), generative graphical models (e.g. Bayesian networks) maintain their benefits even if learned discriminatively and are well-suited for working with missing variables. On the one hand, we have developed methods for generative and discriminative (e.g. max-margin) structure and parameter learning for Bayesian network classifiers. On the other hand, we have applied graphical models to various speech and image processing applications.
Approximate Inference: Performing inference and computing the marginal distributions is a fundamental problem in graphical models. Message passing methods as e.g. belief propagation exploit the structure of the graph and perform approximate inference for models where exact inference is infeasible. Belief propagation works remarkably well in this context and has successfully been applied in many applications. Yet, theoretical results fail to explain this empirical success. We focus on deriving theoretical results that explain how the model specification affects the performance of belief propagation and under which conditions it can be expected to perform well. Moreover, we draw inspiration from our findings and modify the algorithm in ways that improve both the convergence properties and accuracy.