FPGA Implementation of a Neural Network for Radar Interference Mitigation
- Status
- Finished
- Type
- Master Thesis
- Announcement date
- 18 Nov 2020
- Student
- Michael Hirschmugl
- Mentors
- Research Areas
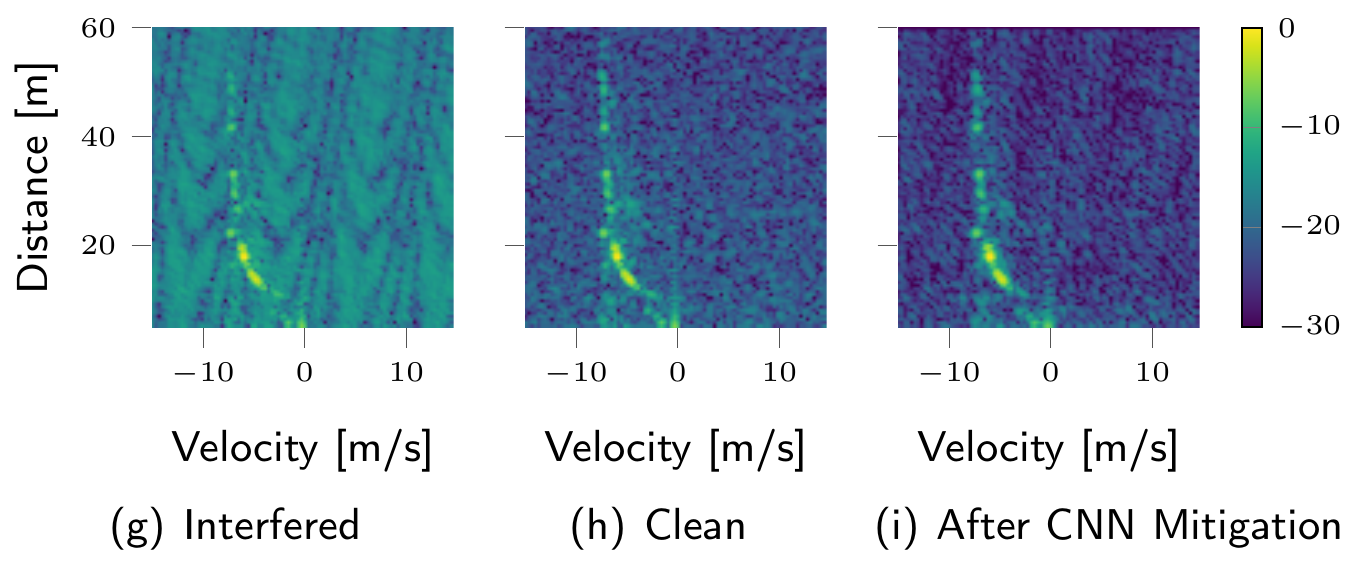
Abstract This thesis proposes a highly optimized convolutional neural network implementation on embedded hardware for radar interference mitigation as a proof-of-concept application in an automotive driver assistance system. The embedded platform is a Xilinx Zynq-7000 system-on-chip, and for resource and performance comparison, the implementation is done in the programmable logic of the device and also as firmware for the integrated processing core. Since interference mitigation based on a neural network, in general, is very expensive in terms of computational efforts and power consumption, different optimization and quantization techniques are investigated and compared. In order to keep implementation and computation as efficient as possible, all floating-point operations are converted to fixed-point and evaluated against the floating-point inference results. In conclusion, all implementations and optimizations are compared in terms of resource utiliza tion in order to be able to select an embedded platform with sufficient resources for a real-world application with specific performance requirements.