Multichannel Voice Activity Detection for ASR
- Status
- Finished
- Type
- Master Thesis
- Announcement date
- 12 Mar 2014
- Student
- Florian Iglisch
- Mentors
- Martin Hagmüller
- Juan Andrés Morales Cordovilla
- Research Areas
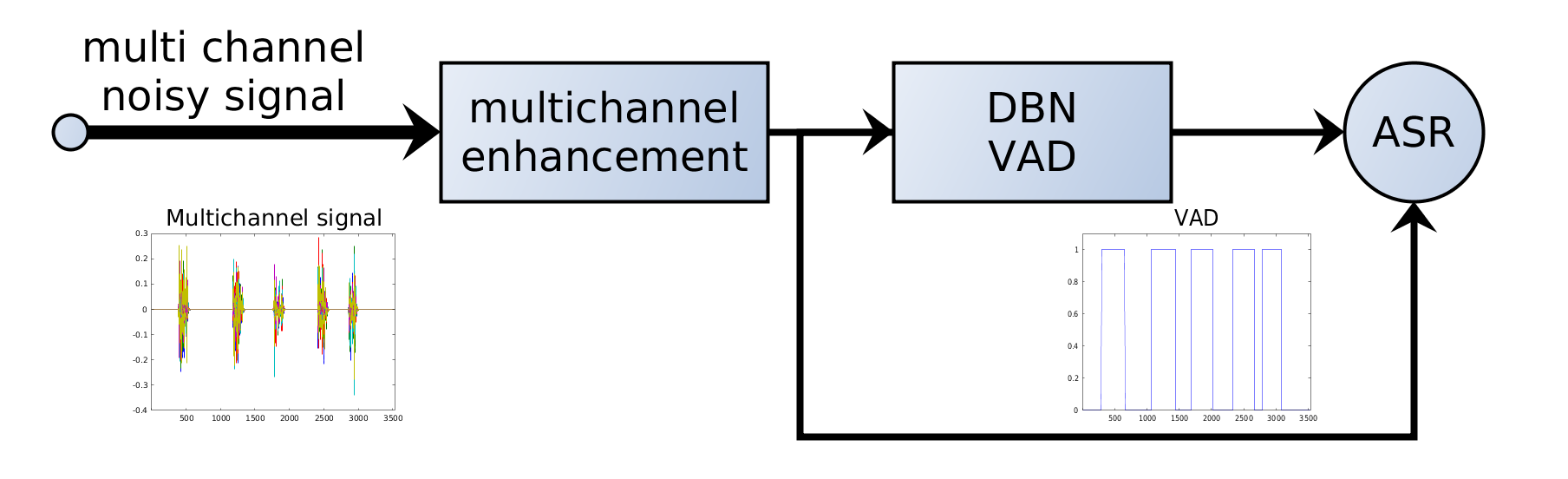
The European Project `Distant-speech Interaction for Robust Home Applications’ (DIRHA) aims to create a system to control a house, using a network of microphones, with German voice commands. Three important parts of this project are: the automatic speech recognizer (ASR), the voice activity detector (VAD) and the speech localizer (SLOC). The Deep-Belief-Network VAD (DBN-VAD) [Zhang2013] is a state-of-the-art VAD and has the ability of fussing many features (pitch, spectrogram, AMS, etc.) to decide if a frame is speech or non-speech. These features can be improved by adding a noise reduction or enhancement stage. For the moment, we are only working with a single-channel signal. The purpose of this master’s thesis is to improve the enhancement, and then the VAD by using spatial information derived from the multi-channel signal [Habib2013]. The final aim is the fusion of the voice activity detector with the source localizer using a pyschoacustical approach [Ma2012].
Your Tasks
- Study literature
- Obtain baseline results with current VAD and ASR on a simulated database
- Improve the VAD using multi-channel information
- Implement real-time system in the kitchen-cocktail party room of the SPSC
Your Profile
- Speech Communication 1 & 2
- Interest in speech recognition and source separation inspired in psycho-acoustical approaches
- Matlab
- Unix-Shell, HTK is a plus, but not necessary
References
[Zhang2013] X. Zhang and J. Wu. Deep Belief Networks Based Voice Activity Detection. IEEE Transactions on Audio, Speech, and Language Processing, 2013.
[Habib2013] T. Habib and H. Romsdorfer. Auditory inspired methods for localization of multiple concurrent speakers. Computer Speech & Language, 2013.
[Ma2012] N. Ma, J. Barker, H. Christensen and P. Green. Combining speech fragment decoding and adaptive noise floor modelling, IEEE Transactions on Audio, Speech, and Language Processing, 2012.