ROBUST - Signal processing for robust speech quality (COAST)
- Links
- Period
- 2006 — 2010
- Funding
- COAST - Kompetenznetzwerk für Sprachtechnologie
- Partners
- Verein Fachhochschule Technikum Wien
- Nuance Communications International (formerly known as Philips Speech Recognition Systems)
- SAIL LABS Technology AG
- Research Areas
- Contact
- Members
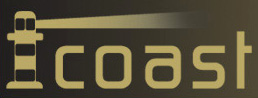
A notorious challenge for automatic speech recognition is the significant decrease of recognition rates encountered under non-ideal acoustic environments. The presence of background noise or of con-current speech from speakers other than the target speaker greatly impairs speech recognition performance. A further obtrusive influence is due to varying recording conditions (diverse noise sources, microphone position, etc.). This base project aims at providing defined and stable signal quality for speech as a precondition for robust speech recognition. This includes the suppression of background noise and of speech of interfering speakers, both being a frequent cause of reduced recognition performance. In addition to noise reduction methods we will primarily investigate new methods for the separation of concurrent acoustic sources, like blind source separation, or, beamforming using multiple microphones. Project targets:
(1) Primary goal is to achieve a defined and stable signal quality for speech - a precondition for robust speech recognition. The speech recognition system should be provided with an enhanced speech signal of the target speaker, even for adverse acoustic environment.
(2) Robust voice activity detection (VAD), to separate speech from background signals.
(3) Blind source separation on one or multiple channels, allowing the division ion of the speech signal of the target speaker from other speech signals or distortions (music, car or machine noise, …)
(4) Source separation using multiple microphone arrays, also for the isolation of the target speaker signal.
(5) The proposed methods aim at the reconstruction of a target speaker signal, that is effected by various acoustic distortions found in real-world conditions, and thus to restore the clean conditions usually met for speech recognizer training.
(6) An important issue for the robustness of the speech recognition system as a whole is the mutual influence between such pre-processing methods and, for example, algorithms for speaker normalization (used to adapt the system to a certain speaker). In this project the interaction between pre-processing algorithms and normalization as well as further subsequent signal processing within state-of-the-art speech recognition systems will also be analyzed.