Uncertainty prediction for prominence classification with chroma features
- Published
- Tue, Apr 01, 2025
- Tags
- rotm
- Contact
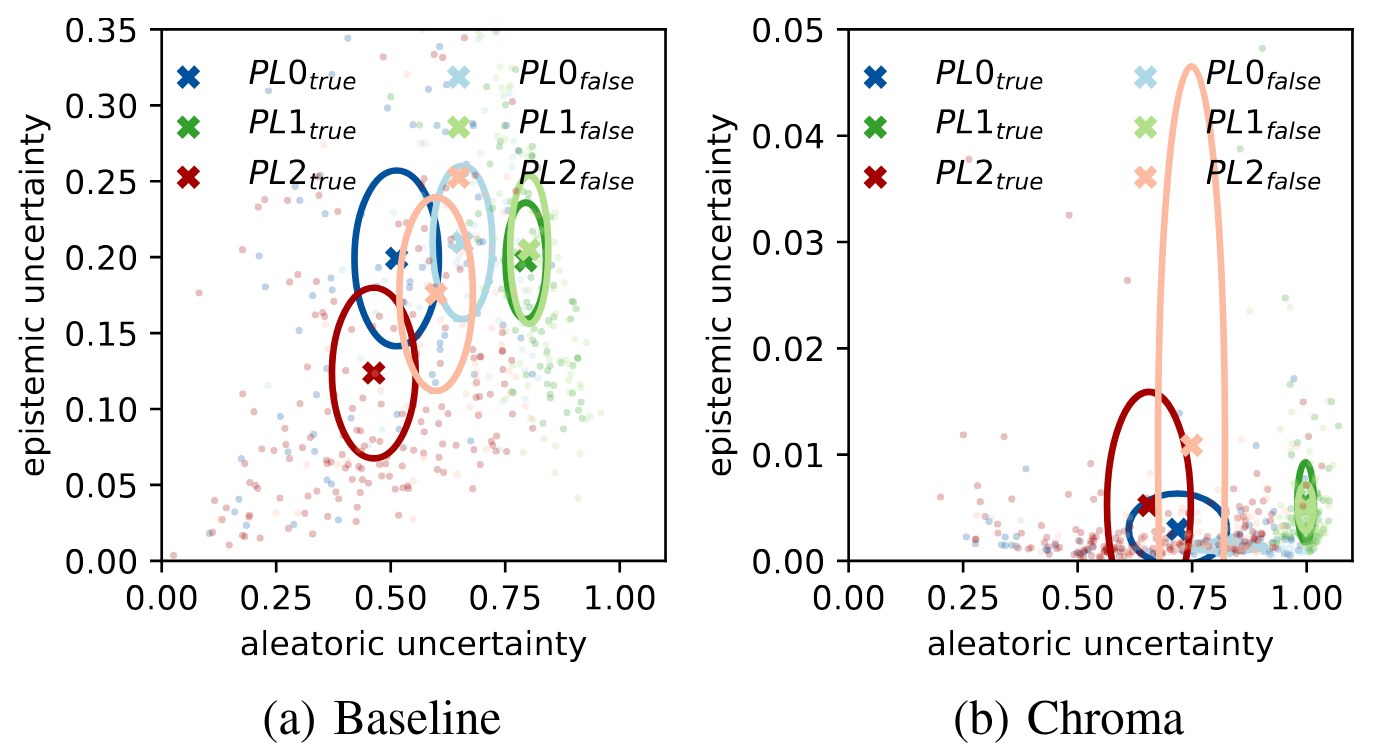
This paper presents methods for prominence classification in conversational speech. Most existing tools rely on prosodic features extracted at syllable- or phone-level, performing well on read speech. This is not the case for conversational speech, where the quality of automatic segmentation is significantly worse. We introduce entropy-based chroma features, requiring only word-level segmentations. They perform equally well as a random forest classifier with prosodic features (requiring phone-level segmentation), with accuracies in the range of the human inter-rater agreement. We further use Bayesian deep learning to quantify the epistemic and aleatoric uncertainty of the prediction for prosodic and chroma features. Whereas the aleatoric uncertainty is, as expected, consistent with inter-rater agreement and similarly high for both feature sets, the epistemic uncertainty is lower for the classifier based on chroma features, indicating higher classification consistency across the corpus.
Browse the Results of the Month archive.