Using Word-Level Features for Prosodic Prominence Classification in Conversational Speech
- Published
- Tue, Aug 01, 2023
- Tags
- rotm
- Contact
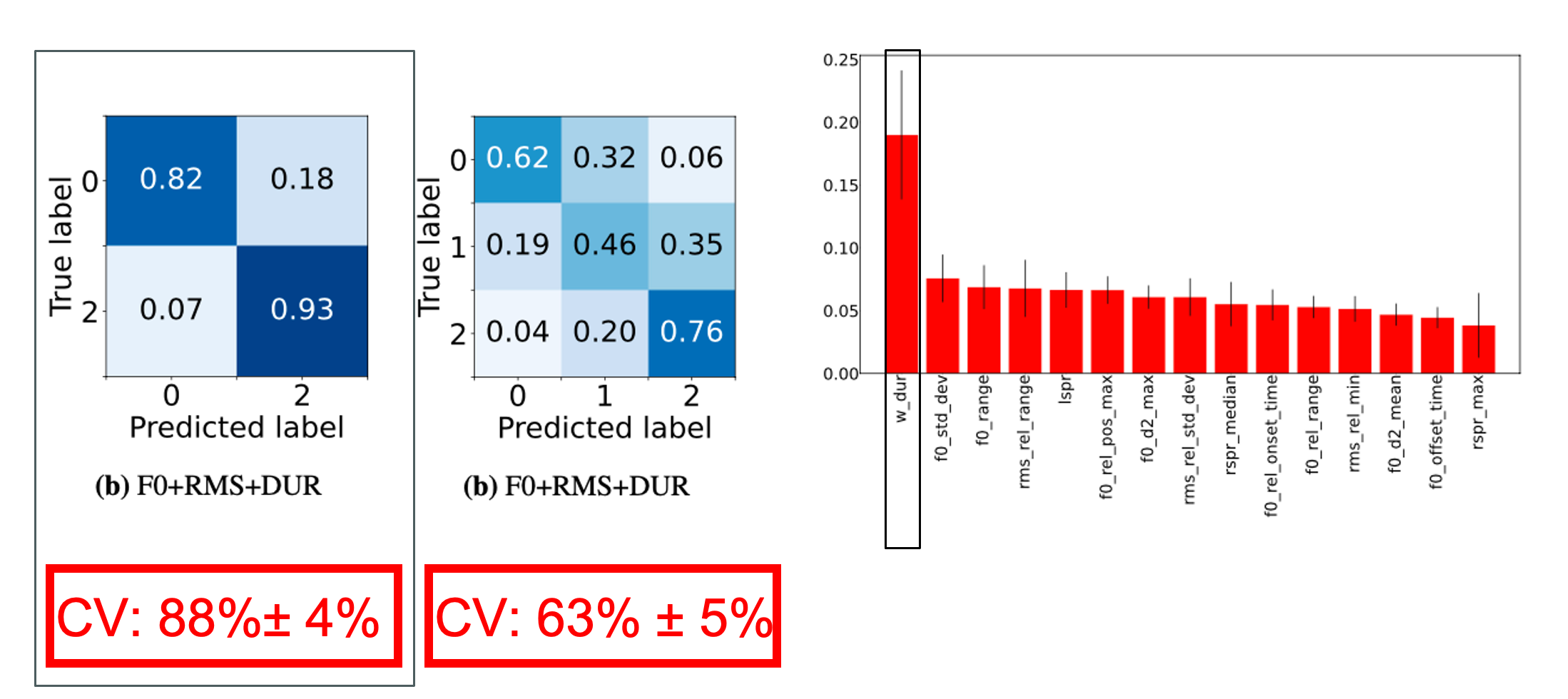
Left: Confusion matrices from experiments with 15 best features (F0+RMS+DUR). Right: Random Forest feature importances for 3 class problem with F0, RMS and DUR.
This work focuses on the automatic detection of prominent words in conversational speech. Most tools for prominence detection rely on prosodic features extracted at a syllable- or phone level and their accuracy thus strongly depends on the quality of the given phone-level segmentation. Given the high degree of pronunciation variation in conversational speech, automatic phonetic segmentation is not accurate enough to detect prominence reliably. Here we explore different approaches to prominence detection that require merely a prior word-level segmentation. The first experiment shows that by using word-level prosodic features cross-validation accuracies of 88%+-4% can be reached, and that word duration is the most important feature. The second experiment introduces entropy-based fundamental frequency and intensity features for prominence detection. Our findings suggest that entropy-based, word-level features can provide a robust approach to detecting prominent words in conversational speech
This work is published at the twentieth edition of ICPhS and will be presented in August 2023.
Browse the Results of the Month archive.