Conversational Speech Recognition Needs Data? Experiments with Austrian German
- Published
- Wed, Jun 01, 2022
- Tags
- rotm
- Contact
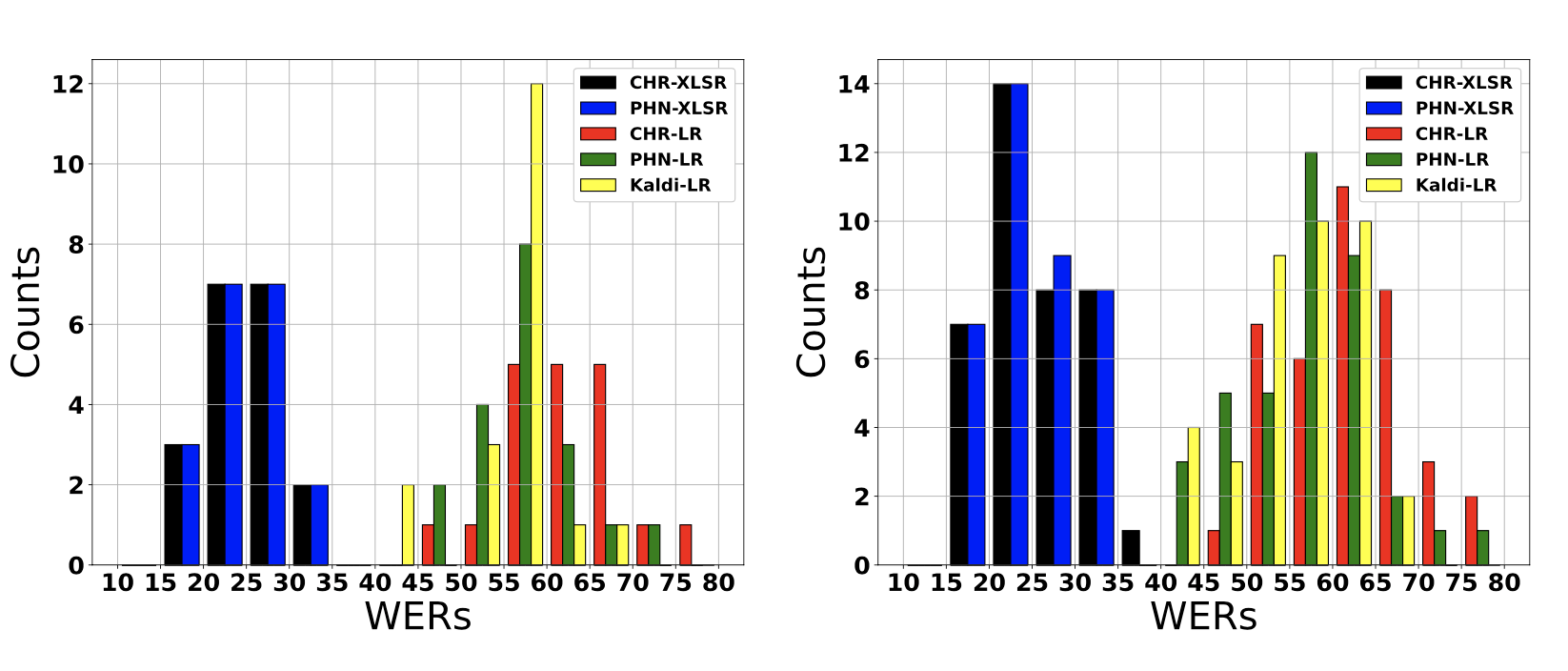
Left: Histogram showing conversation-dependent WERs of low-resource (LR) and data-driven (XLSR) 4-gram models. Right: Histogram showing speaker-dependent WERs of low-resource (LR) and data-driven (XLSR) 4-gram models.
We show that data-driven speech recognition systems are effective for Austrian German conversational speech but we still observe a lack of robustness to inter-speaker and inter-conversation variation.
Low-resource (LR) speech recognition is challenging since two humans who interact spontaneously with each other introduce complex inter- and intra-speaker variation depending on for instance the speaker’s attitude towards the listener and the speaking task. Recent developments in self-supervision have allowed LR-scenarios to take advantage of large amounts of otherwise unrelated data. In this study, we characterize an (LR) Austrian German conversational task. We begin with a non-pre-trained baseline (Kaldi-LR) and show that fine-tuning of a model pre-trained using self-supervision (XLSR) leads to improvements consistent with those in the literature; this extends to cases where a lexicon and language model are included. Further, by use of leave-one-conversation out technique, we demonstrate that robustness problems remain with respect to inter-speaker and inter-conversation variation. This serves to guide where future research might best be focused in light of the current state-of-the-art.
This work is published at the thirteenth edition of LREC and will be presented in June 2022.
Browse the Results of the Month archive.