Heart Sound Segmentation—An Event Detection Approach Using Deep Recurrent Neural Networks
- Published
- Sat, Sep 01, 2018
- Tags
- rotm
- Contact
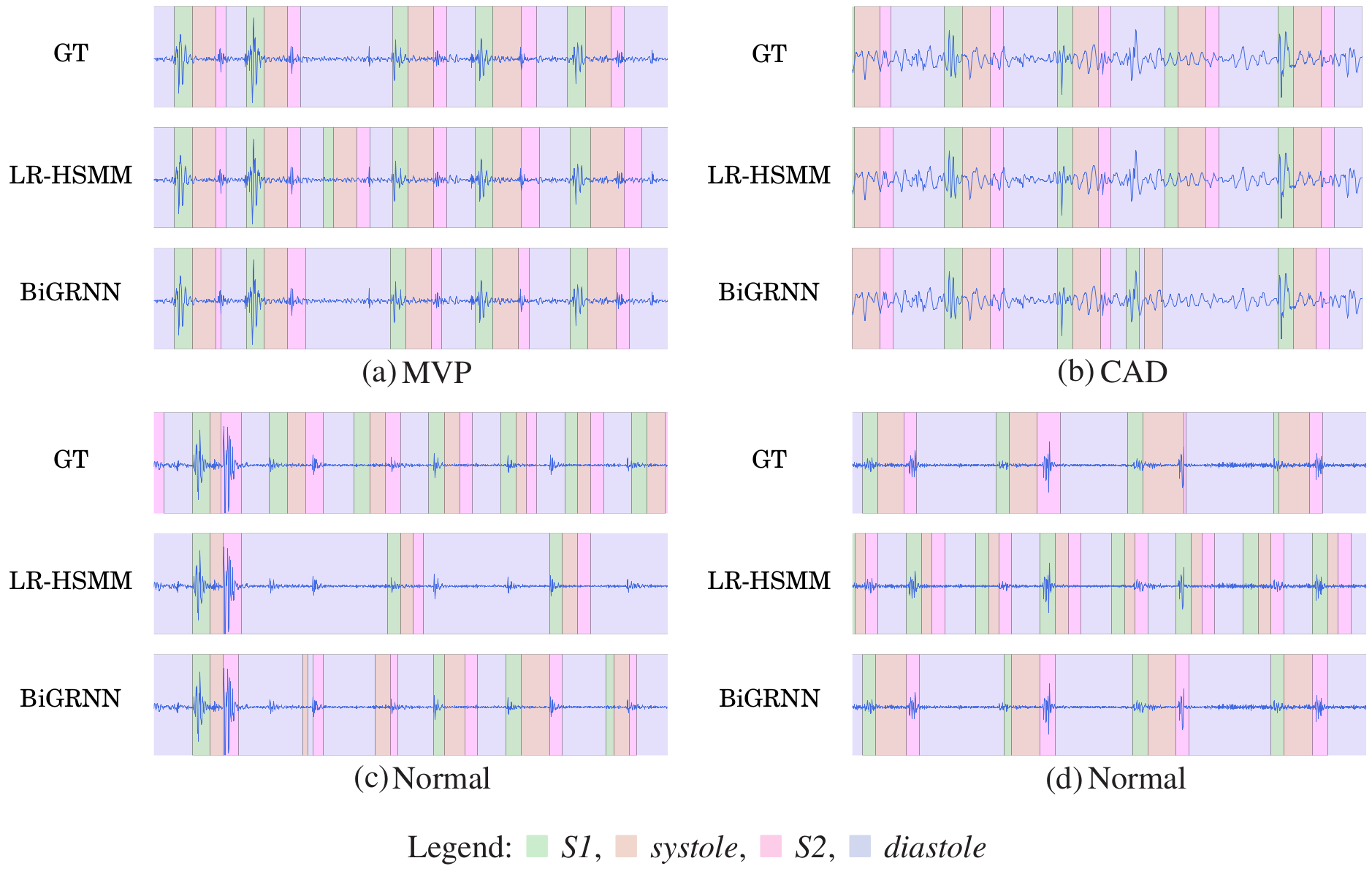
We present a method to accurately detect the state-sequence first heart sound (S1)–systole–second heart sound (S2)–diastole , i.e., the positions of S1 and S2, in heart sound recordings. We propose an event detection approach without explicitly incorporating a priori information of the state duration. This renders it also applicable to recordings with cardiac arrhythmia and extendable to the detection of extra heart sounds (third and fourth heart sound), heart murmurs, as well as other acoustic events. We use data from the 2016 PhysioNet/CinC Challenge, containing heart sound recordings and annotations of the heart sound states. From the recordings, we extract spectral and envelope features and investigate the performance of different deep recurrent neural network (DRNN) architectures to detect the state sequence. We use virtual adversarial training, dropout, and data augmentation for regularization. We compare our results with the state-of-the-art method and achieve an average score for the four events of the state sequence of F1≈96% on an independent test set.
The figure shows examples of automatically segmented heart sound recordings (snippets of four seconds each). In each subfigure, the first plot corresponds to the hand annotated ground truth (GT), the second to the logistic regression hidden semi-Markov model based (LR-HSMM) method and the third to our proposed method (BiGRNN). The recordings are from normal subjects and pathological patients: Normal control group (Normal), coronary artery disease (CAD), and murmurs related to mitral valve prolapse (MVP).
More information can be found in our paper.
Browse the Results of the Month archive.