Eigenvector-based Speech Mask Estimation for Multi-Channel Speech Enhancement
- Published
- Mon, May 01, 2017
- Tags
- rotm
- Contact
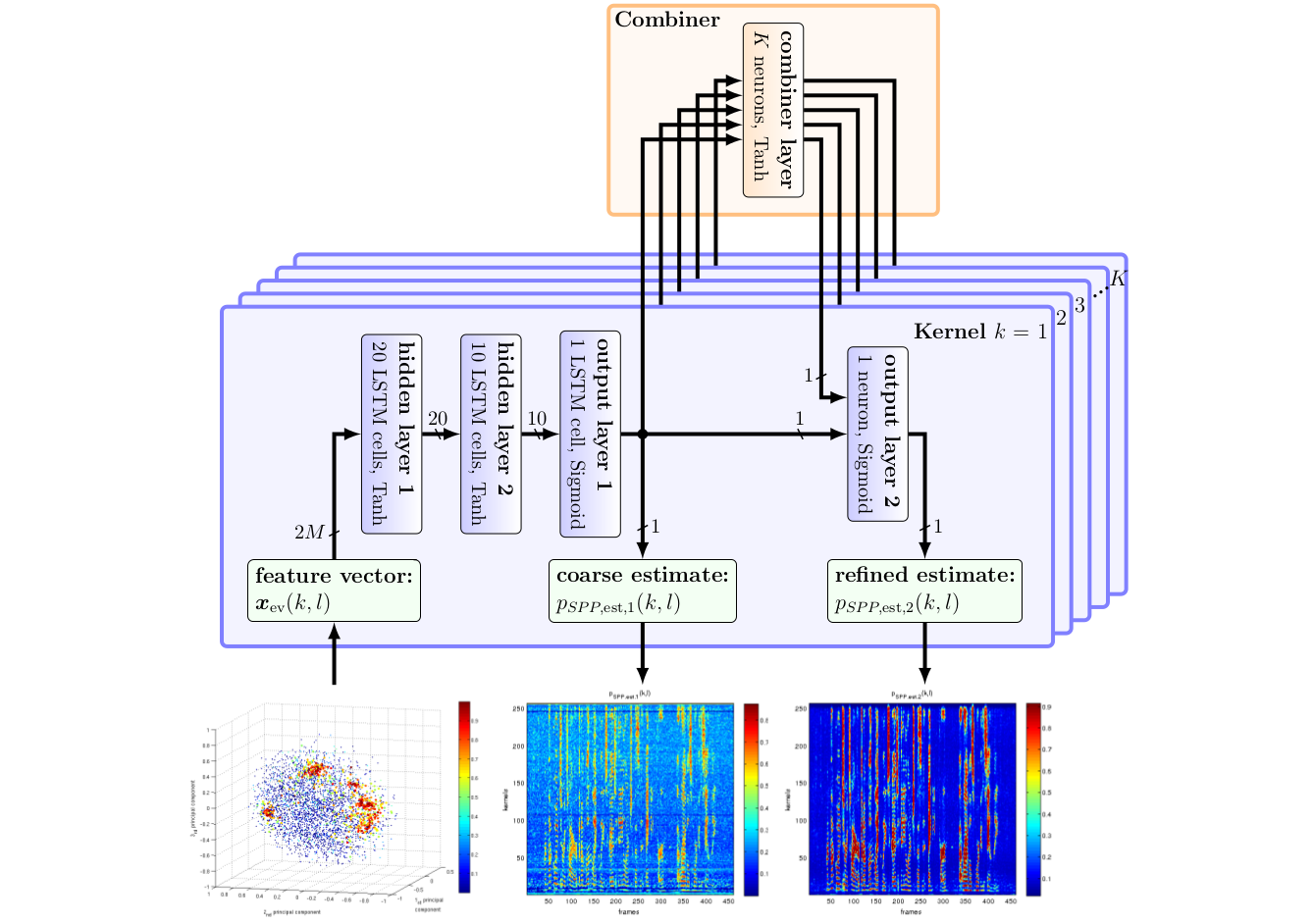
Using speech masks for multi-channel speech enhancement gained attention over the last years, as it combines the benefits of digital signal processing (beamforming) and machine-learning (learn the speech mask from data). We demonstrate how a speech mask can be used to construct the Minimum Variance Distortionless response (MVDR), Generalized Sidelobe Canceler (GSC) and Generalized Eigenvalue (GEV) beamformers, and a MSE-optimal postfilter. We propose a neural network architecture that learns the speech mask from the spatial information hidden in the multi-channel input data, by using the dominant eigenvector of the Power Spectral Density (PSD) matrix of the noisy speech signal as feature vector. We use CHiME-4 audio data to train our network, which contains a single speaker engulfed in ambient noise. Depending on the speakers location and the geometry of the microphone array the eigenvectors form local clusters, whereas they are randomly distributed for the ambient noise. The neural network learns this clustering from the training data. In a second step, we use the cosine similarity between neighboring eigenvectors as feature vector, which makes our approach less dependent on the array geometry and the speaker’s position. We compare our results against the most prominent model-based and data-driven approaches, using PESQ and PEASS/OPS scores. Our system yields superior results, both in terms of perceptual speech quality and speech mask prediction error.
The figure gives an overview of the neural network we used to estimate the speech mask. The network uses the dominant eigenvector of the noisy speech PSD as feature vector, as indicated in the lower left corner. For each frequency bin k, a small kernel is used to produce a coarse estimate pSPP,est,1. Each kernel is shown as blue rectangle, consisting of 2 hidden layers with 20 and 10 LSTM cells, respectively. The coarse estimates from all frequency bins are then fed into the combiner layer, which is shown at the very top in the orange rectangle. By combining all predictions from all kernels, the combiner exploits the broadband nature of human speech, which produces a second refined estimate pSPP,est,2.
More information can be found in our paper.
Browse the Results of the Month archive.