Multichannel speech processing architectures for noise robust speech recognition: 3rd CHiME Challenge results
- Published
- Mon, Feb 01, 2016
- Tags
- rotm
- Contact
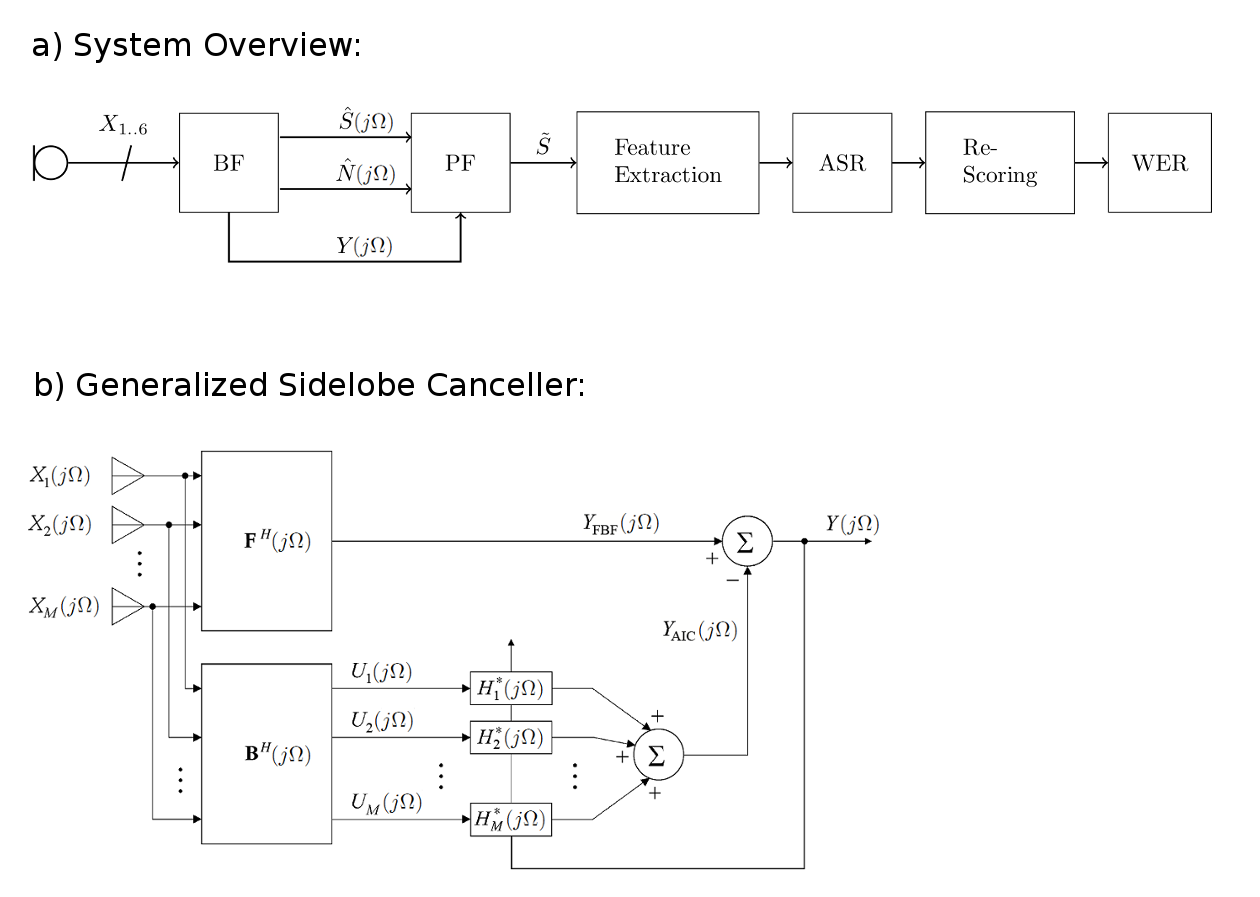
Recognizing speech under noisy condition is an ill-posed problem. The CHiME3 challenge targets robust speech recognition in realistic environments such as street, bus, caffee and pedestrian areas. We study variants of beamformers used for pre-processing multi-channel speech recordings. In particular, we investigate three variants of generalized sidelobe canceller (GSC) beamformers, i.e. GSC with sparse blocking matrix (BM), GSC with adaptive BM (ABM), and GSC with minimum variance distortionless response (MVDR) and ABM. Furthermore, we apply several postfilters to further enhance the speech signal. We introduce MaxPower postfilters and deep neural postfilters (DPFs). DPFs outperformed our baseline systems significantly when measuring the overall perceptual score (OPS) and the perceptual evaluation of speech quality (PESQ). In particular DPFs achieved an average relative improvement of $17.54% OPS points and $18.28% in PESQ, when compared to the CHiME3 baseline. DPFs also achieved the best WER when combined with an ASR engine on simulated development and evaluation data, i.e. 8.98% and 10.82% WER. The proposed MaxPower beamformer achieved the best overall WER on CHiME3 real development and evaluation data, i.e. 14.23% and 22.12%, respectively.
An overview of our speech enhancement and recognition system is given in a). The BF block denotes a GSC beamformer, which feeds three signals into the multi-channel postfilter (PF), which are the speech and noise estimates S^ and N^, and the beamformer output Y. The postfilter produces a speech estimate S~, which is used to extract MFCC and PNCC feature. These feature vectors are fed into the Kaldi ASR subsystem. The rescoring mechanism employs a recurrent neural network language model (RNN-LM), which is trained on the provided Wall Street Journal speech corpus (WSJ0). The final block evaluates the word-error rate (WER) in percent.
The GSC beamformer is shown in greater detail in b). It is comprised of a fixed beamforming vector F, a blocking matrix B, and an adaptive interference canceller H. The speech and noise estimates S^ and N^ are produced by back-projecting the output Y to the inputs X using the speaker location.
More information can be found in our paper!
Browse the Results of the Month archive.