General Stochastic Networks for Classification
- Published
- Mon, Dec 01, 2014
- Tags
- rotm
- Contact
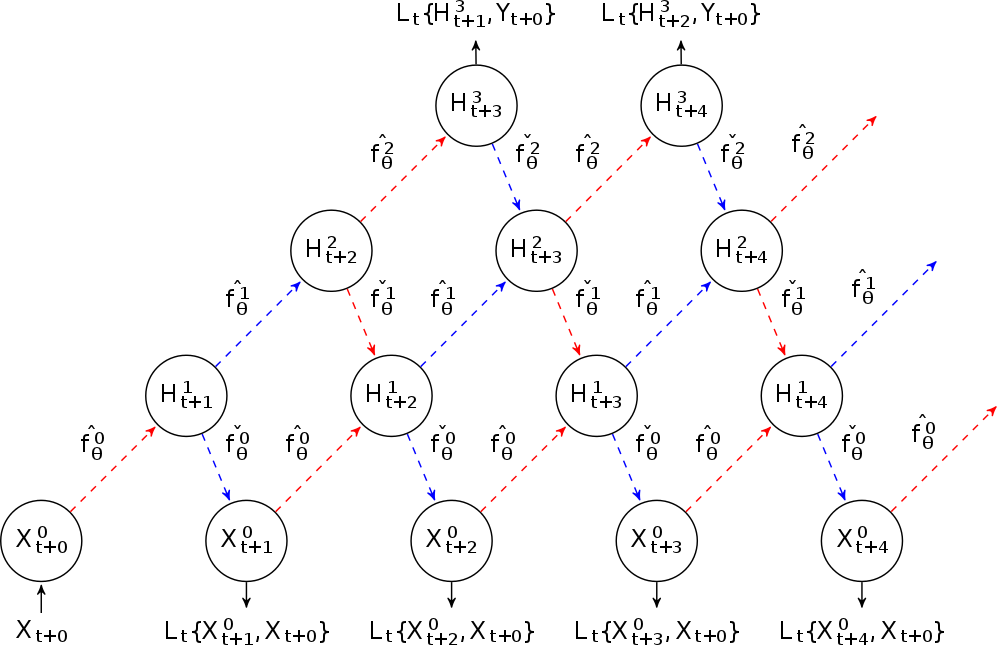
We extend generative stochastic networks to supervised learning of representations. In particular, we introduce a hybrid training objective considering a generative and discriminative cost function governed by a trade-off parameter λ. We use a new variant of network training involving noise injection, i.e. walkback training, to jointly optimize multiple network layers. Neither additional regularization constraints, such as 1, 2 norms or dropout variants, nor pooling- or convolutional layers were added. Nevertheless, we are able to obtain state-of-the-art performance on the MNIST dataset, without using permutation invariant digits and outperform baseline models on sub-variants of the MNIST and rectangles dataset significantly.
The figure shows a GSN Markov chain for input Xt+0 and target Yt+0 with backprop-able stochastic units.
Browse the Results of the Month archive.