ASR for Electro-Laryngeal Speech
- Published
- Sun, Sep 01, 2013
- Tags
- rotm
- Contact
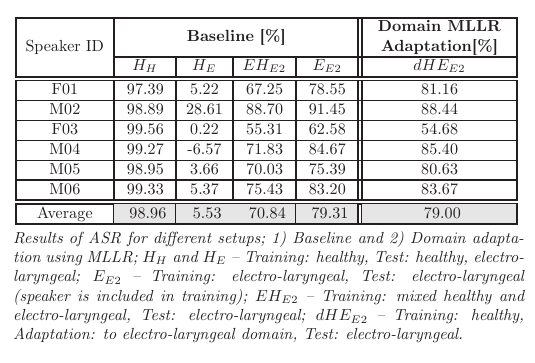
In this work we apply disordered speech, namely speech produced by an Electro-Larynx (EL), on an Automatic Speech Recognition (ASR) system which was designed for normal, healthy speech. When disordered speech is applied to ASR systems, the performance will significantly decrease. ASR systems are increasingly becoming part of daily life. Therefore, the word accuracy rate of disordered speech should be reasonably high to make ASR technologies accessible for patients suffering from speech disorders.
Experiments: In the table, word accuracy (WAcc) rates are shown for different setups. The WAcc, when training material only consists of healthy speech and we test on healthy speech, is 98.96% (Baseline - HH). When the test is carried out on electro-laryngeal speech, the performance is very low (5.53%; Baseline - HE) due to the mismatched domain. When speech material of electro-laryngeal speech is added to the healthy training, (EHE2) the word accuracy rate improves to 70.84% regarding HE. The results improve even further using only electro-laryngeal speech for training (79.31%; Baseline - EE2).
In the next experiment we apply domain MLLR adaptation of healthy speech to electro-laryngeal speech to investigate the case when only little data is available. With this approach we reach a word accuracy of 79.00%.
Conclusion: These results show that, although there is a large mismatch between the two domains, as soon as we include electro-laryngeal speech in the training, the ASR system performs well. Connected to that we also have shown that it is possible to obtain a robust EL model starting from a healthy speech model. This is important because state-of-the-art systems train triphone models and for this reason large amounts of speech material are needed.
For more information please refer to our paper “ASR for Electro-Larynx Speech”.
Browse the Results of the Month archive.