Speech Enhancement Using Pre-Image Iterations
- Published
- Sun, Apr 01, 2012
- Tags
- rotm
- Contact
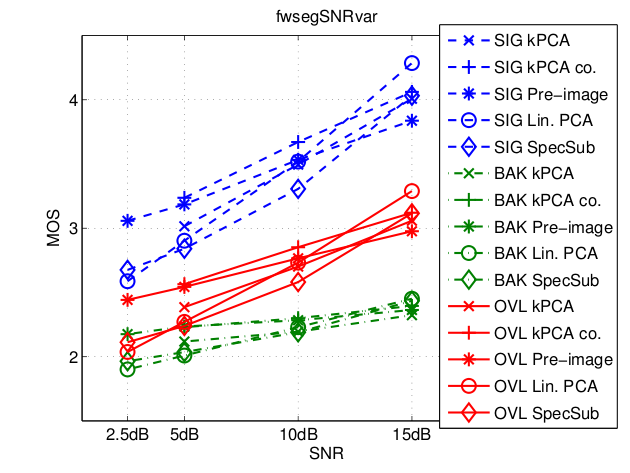
In this work, we show how to de-noise speech in the complex spectral domain using pre-image iterations. The method is derived from kernel principal component analysis (kPCA). Instead of applying PCA in a high-dimensional feature space and then going back to the original input space by using a solution to the pre-image problem, only the pre-image step is applied for de-noising. We show that the de-noised audio sample is a convex combination of the noisy input data and that the resulting algorithm is closely related to the soft k-means algorithm. Compared to kPCA, this method reduces the computational costs while the audio quality is similar and speech quality measures do not degrade.
The figure presents a comparison of the results from pre-image iterations (Pre-image) to the results of kernel PCA (kPCA), kernel PCA with combined pre-imaging (kPCA co.), linear PCA (Lin. PCA), and spectral subtraction (SpecSub) using a variant of the frequency-weighted SNR that separatly evaluates the signal quality (SIG), the background intrusion (BAK), and the overall quality (OVL) and returns a mean opinion score (MOS), where high values denote better quality. The pre-image iteration method achieves scores comparable to the other methods, for low SNRs it even outperforms most of them.
More details are described in our paper that was presented at ICASSP 2012.
Browse the Results of the Month archive.