Classification of homophones in conversational Austrian German via Random Forest
- Status
- Finished
- Type
- Bachelor Project
- Announcement date
- 10 Aug 2020
- Student
- Xenia Kogler
- Mentors
- Research Areas
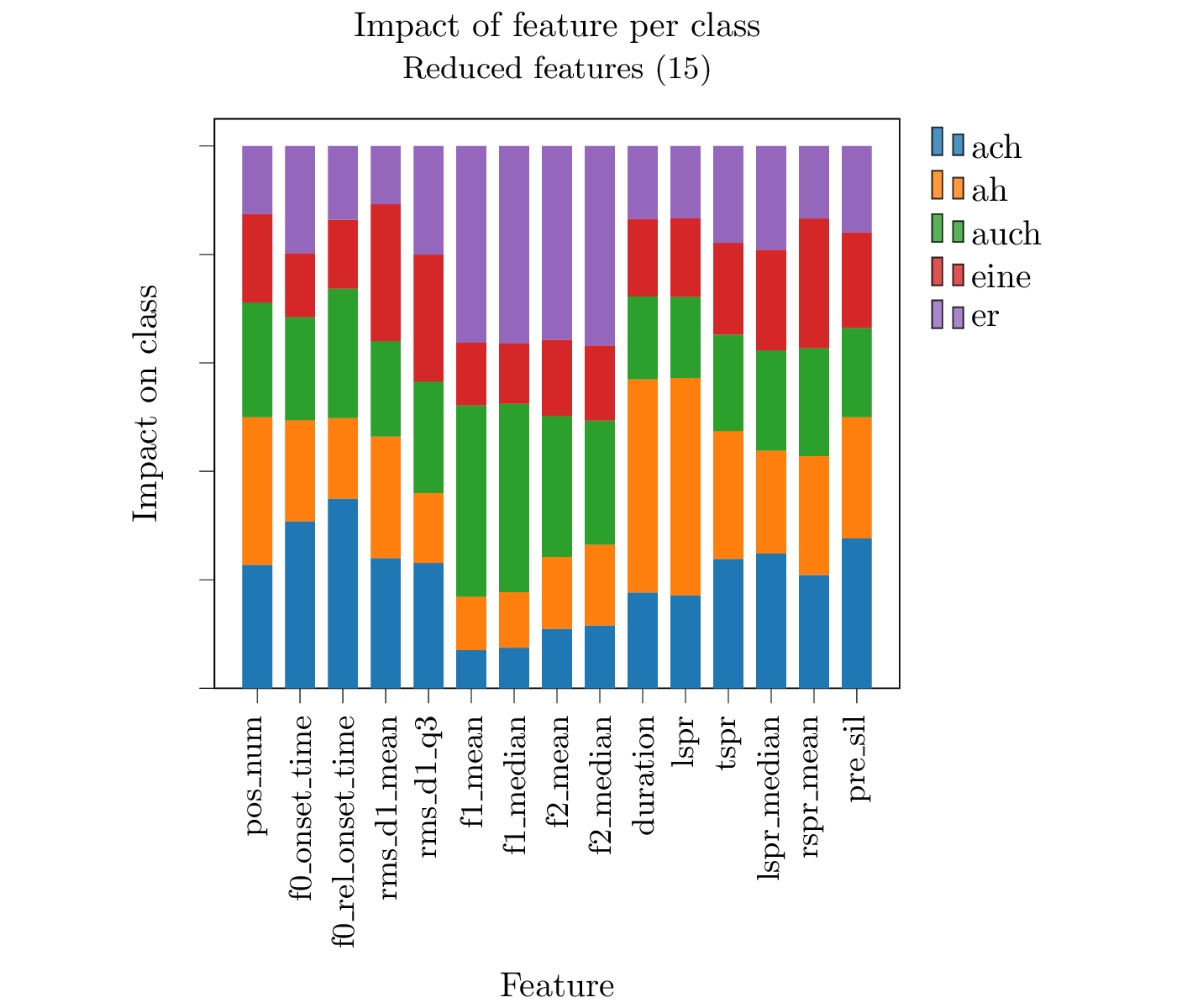
This thesis aimed at gaining a better understanding of the acoustic features of homophones, especially regarding spontaneous speech in Austrian German. I posed the question if homophones are acoustically identical or if there are acoustic cues that might give information about the meaning of the word. The data was extracted from the Conversational Speech Component (CSC) of the GRASS corpus. From 4174 tokens of the word types ‘ach’, ‘ah’, ‘auch’, ‘eine’ and ‘er’, I extracted 128 acoustic features (f0, RMS, durational and spectral features). I used a Random Forest to perform a classification of the tokens and calculated the feature importance to reduce the features to the most important ones. Using SHAP values I calculated the impact of the features on the five classes as shown in the figure. It shows that the durational features have a great impact on the class ‘ah’, f0 impacts the class ‘ach’, whereas the spectral features have a bigger impact on the classes ‘er’ and ‘auch’.
The model trained on acoustic features achieved promising results. With only 15 features the performance of the model for the five classes was quite good. The F1-score of the classification was 51%, the Out-of-Bag-score (OOB) was 53% and the accuracy 54%. F1-score and accuracy were consistantly higher than chance, except for the class ‘eine’. The figure shows that non of the important features has a specific impact on that class. Improving the feature selection and reducing class confusability could be goals for refining this work. There was not a very high number of tokens, so increasing the data size could also increase performance. The experiments done in this study, show that a classification of homophones in spontaneous speech using acoustic cues works reasonably well and that this is a promising approach towards decreasing word error rates in automatic speech recognition (ASR).