Creating a new combined confidence measure for ASR-errors on the word-level
- Status
- Finished
- Type
- Master Thesis
- Announcement date
- 01 Oct 2012
- Student
- Philipp Salletmayr
- Mentors
- Research Areas
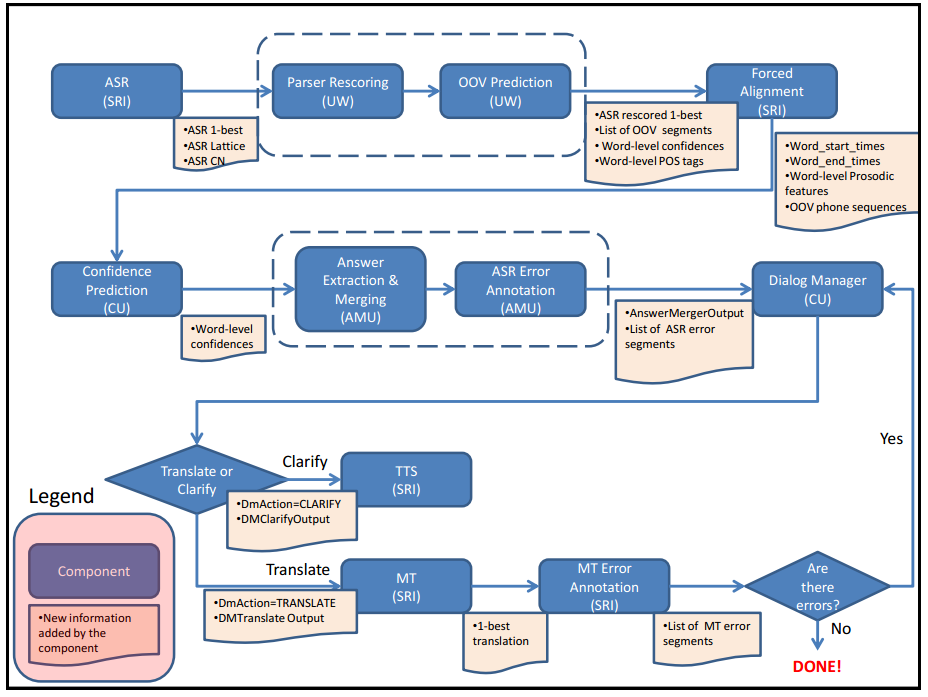
Abstract
Most current dialog systems employ very simple strategies when dealing with misrecognitions i.e. “please repeat/rephrase”. This causes problems for the user, as it is not known what exact part of an utterance was misrecognized. This thesis addresses the problem of localized error detection in Automatic Speech Recognition (ASR) output, seeking to identify which particular words in an utterance have been misrecognized. Identifying misrecognized words permits one to create targeted clari cation strategies for spoken dialogue systems, allowing the system to ask clari cation questions targeting the particular type of misrecognition. This thesis presents results from machine learning experiments using ASR con dence scores together with prosodic and syntactic features to predict (1) whether an utterance contains an error, and (2) what exact word(s) in a misrecognized utterance are misrecognized. Experiments conducted using di erent classi cation techniques on the TRANSTAC database showed, that by adding prosodic and syntactic features to the ASR features, prediction of misrecognized utterances improves compared to using ASR features alone. This means that an interactive system with clari cation capabilities using the proposed error detection method would attempt to correct over half of misrecognized words with a clarification subdialogue. These findings are used to build a classi er for an error detection module in a Spoken Dialog System (SDS).