Classifying the meaning of breathings in conversational speech
- Status
- Finished
- Type
- Master Thesis
- Announcement date
- 08 Oct 2019
- Student
- André Menrath
- Mentors
- Research Areas
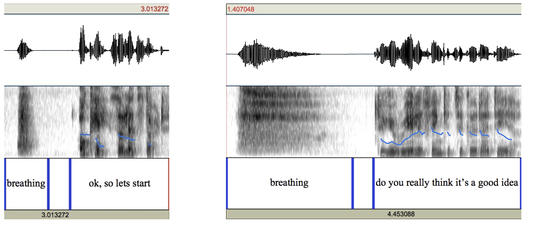
Automatic speech recognition (ASR) systems were originally designed to cope with carefully pronounced speech. Most real world applications of ASR systems, however, require the recognition of spontaneous, conversational speech (e.g., dialogue systems, voice input aids for physically disabled, medical dictation systems, etc.). Compared to prepared speech, conversational speech contains utterances that might be considered ‘ungrammatical’ and contain disfluencies such as “…oh, well, I think ahm exactly …”. Moreover, in spontaneous conversation, people do not only breathe (silently) with a physical function but also clearly audible with a communicative function. In the following sentence the speaker produces a long breathing before speaking:
” …. do you really think this is a good idea?” communicative fuction: doubting
whereas in the following sentence, the speaker produces a short strong breathing before speaking:
“…. ok, so le’ts start, we don’t have much time left!” communicative function: starting an action
This master thesis aims to deepen our understanding of the impact of audible breathing on turn-taking in conversation. It provides an overview of the contexts in which audible breathings occur and aims to identify features of breath noises pertinent to their communicative function. This is based on the hypothesis that breathings are a practice that can be shaped by the speaker. We conducted quantitative analyses using the Graz Corpus of Read and Spontaneous Speech (GRASS), where audible breath sounds were manually annotated. We extracted contextual, durational, and acoustic features of annotated audible breathings and employed classification algorithms to predict surrounding turn-taking labels, which were derived from annotations of Points of Potential Completion (PCOMP). These offered a fine-grained representation of the conversation, as they point out each turn-relevance place in time (TRP). For the classification tasks, we separately employed a random forest and two gradient boosting machine learning models. The model with the highest Matthews correlation coefficient (MCC) score was selected for the subsequent analysis. This involved the use of SHAP (SHapley Additive exPlanations) values to determine the importance and impact of the features. Firstly, the analysis highlighted the relation of contextual and durational features of audible breathing to turn-taking. This was used as a foundation for investigating the further impact of acoustic features, with a particular focus on those that have been proposed in the literature (e.g., the relative intensity). Additionally, the identification of further acoustic features was of interest. Our analysis demonstrated that features that are most likely related to the audibility of breathings, emerged as promising predictors of the speaker taking the turn. On the other end, backchannels (i.e., hearer-response tokens) were more frequently associated with residual breaths or longer inhalation phases. The findings support existing research and intuitive expectations, though the prediction scores were lower than anticipated, likely due to the highly spontaneous nature of the employed speech data. However, this thesis also points out methodological limitations in interpreting acoustic features, and thus establishes a foundation for future research on the relationship between audible breathing and turn-taking in conversation.