Neural-Enhanced Multipath-based SLAM
- Status
- Open
- Type
- Master Thesis
- Announcement date
- 22 Mar 2022
- Mentors
- Research Areas
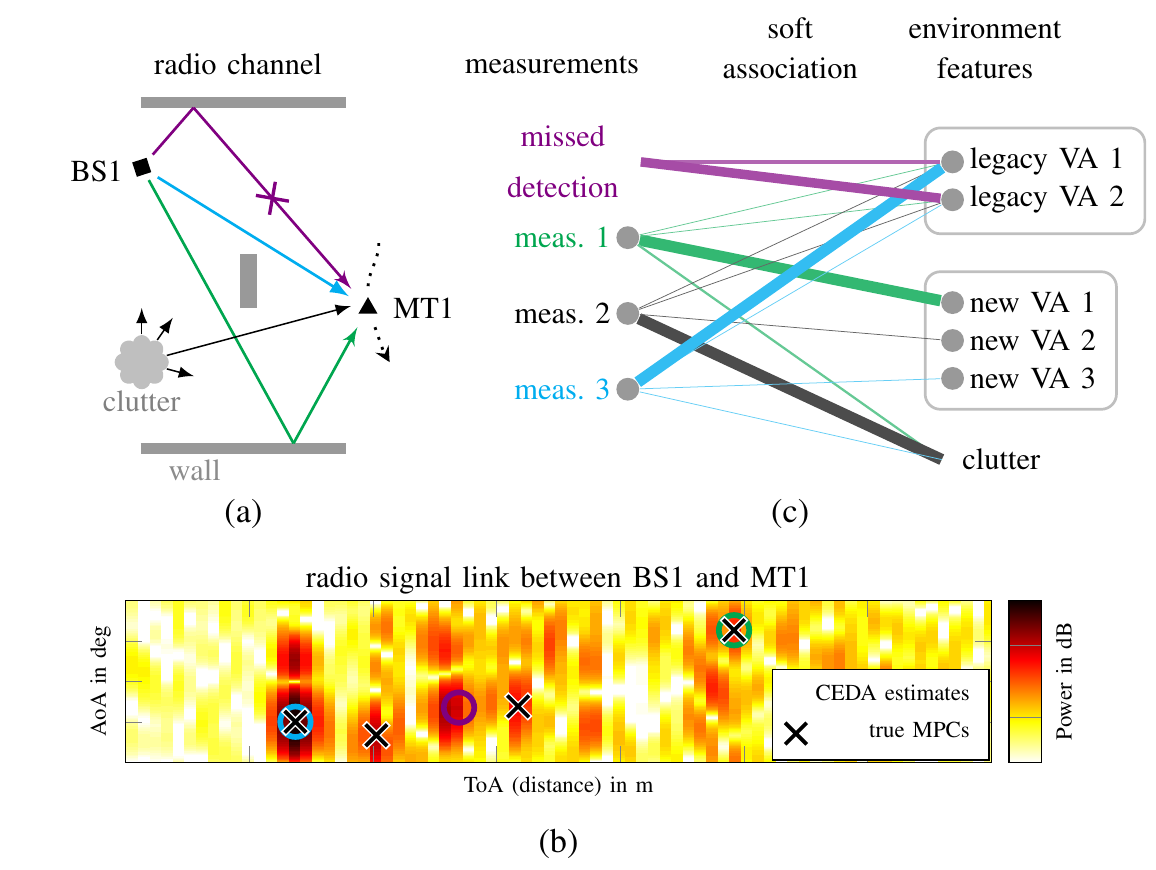
Description
Accurate indoor localization using radio signals exchanged between base stations (BSs) and mobile agents (such as mobile phones and other radio-equipped devices) remains a challenging issue. This capability is essential for various critical applications, including search-and-rescue operations and autonomous navigation. Simultaneous Localization and Mapping (SLAM) involves constructing a feature map of an environment while simultaneously estimating the location of an agent within that map. A promising approach in this domain is multipath-based SLAM, which leverages the specular reflections of radio signals from flat surfaces to estimate the positions of both a mobile agent and the environmental features (e.g., reflecting surfaces). Current model-based techniques for multipath-based SLAM employ high-dimensional Bayesian estimation methods using factor graphs [1]. However, these techniques rely on parametric models, making them vulnerable to performance degradation when the assumptions about feature models or statistical characteristics do not align with actual data. To address this limitation, the parametric structure of the factor graph can be augmented with neural networks, enabling the model to adapt to data-driven insights and correct for model mismatches [2,3].
References
- [1] E. Leitinger, F. Meyer, F. Hlawatsch, K. Witrisal, F. Tufvesson, and M. Z. Win, “A belief propagation algorithm for multipath-based SLAM,” vol. 18, no. 12, pp. 5613–5629, Dec. 2019.
- [2] A. Venus, E. Leitinger, S. Tertinek, and K. Witrisal, “A neural-enhanced factor graph-based algorithm for robust positioning in obstructed LOS situations,” IEEE Open J. of Signal Process., vol. 5, pp. 29–38, Nov. 2023.
- [3] S. Wei, M. Liang, and F. Meyer, “A new architecture for neural enhanced multiobject tracking,” in Proc. FUSION 2024, Jul. 2024, pp. 1–8.
Your Tasks:
- Conduct literature research on neural-enhanced (hybrid) graph-based methods.
- Using [1] as a foundation, develop factor graph structures that integrate neural networks as replacements for traditional factors.
- Evaluate performance on both synthetic (provided simulation environment) and real-world datasets.
What we expect from you:
- Familiar with (statistical) signal processing.
- Inclined to do theoretical/simulation based work.
- Some experience with python and matlab is beneficial.
Contact
If you are interested and want to know more about it, send me an email to erik.leitinger@tugraz.at.