Explainable machine learning for breakdown prediction in high gradient rf cavities
- Published
- Tue, Nov 01, 2022
- Tags
- rotm
- Contact
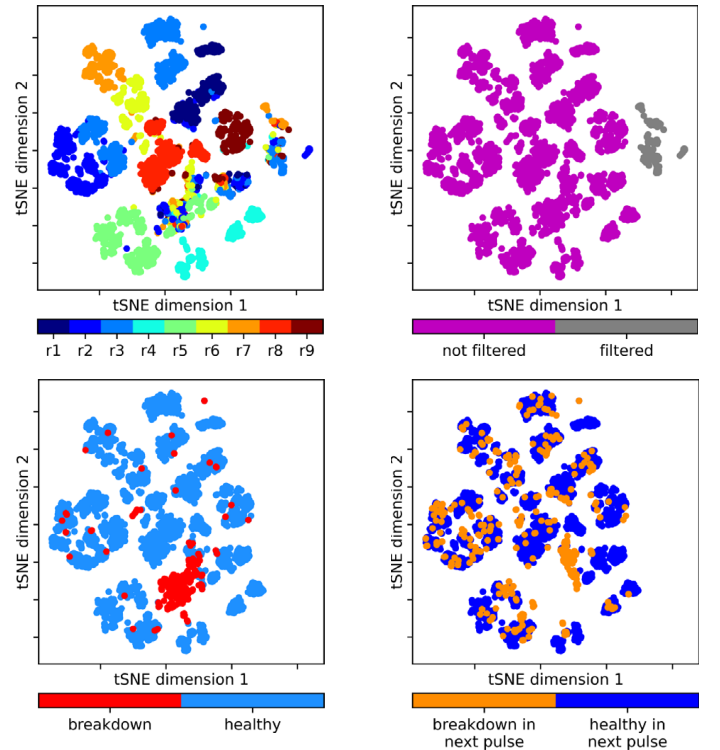
In our latest project with CERN, we used machine learning to analyze breakdowns in a test bench for the CLIC accelerator. In particle accelerators, one of the most prevalent limits on high-gradient operation is the occurrence of vacuum arcs, commonly known as radio frequency (RF) breakdowns. During a breakdown, field enhancement, associated with small deformations on the cavity surface, results in electrical arcs which may irreparably damage the RF cavity surface. In the project, supervised and unsupervised methods were used for data analysis and a breakdown prediction study. ‘Explainable-AI’ made it possible to interpret learned model parameters and to reverse engineer physical properties in the test bench. Similar models could be applied to cancer treatment, light sources, and CERN next generation high energy physics facilities.
The work was recently published in the Journal of Physical Review Accelerators and Beams (PRAB) and is available here.
Browse the Results of the Month archive.