Deep Convolutional Neural Networks for Massive MIMO Fingerprint-Based Positioning
- Published
- Sun, Aug 01, 2021
- Tags
- rotm
- Contact
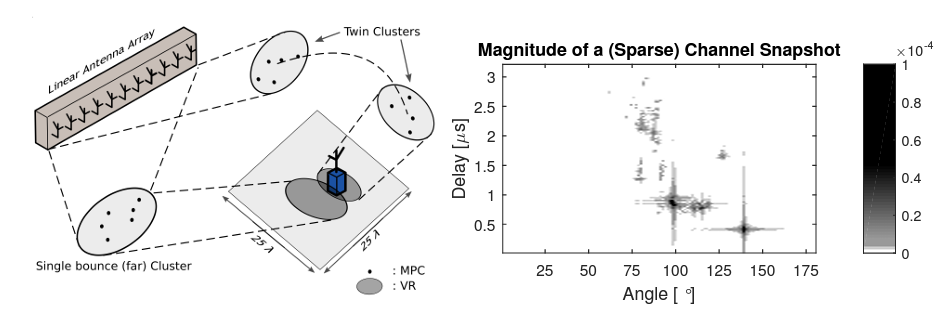
This work provides an initial investigation on the application of convolutional neural networks (CNNs) for fingerprint-based positioning using measured massive MIMO channels. When represented in appropriate domains, massive MIMO channels have a sparse structure which can be efficiently learned by CNNs for positioning purposes. We evaluate the positioning accuracy of state-of-the-art CNNs with channel fingerprints generated from a channel model with a rich clustered structure: the COST 2100 channel model. We find that moderately deep CNNs can achieve fractional-wavelength positioning accuracies, provided that an enough representative data set is available for training.
The full version of this paper can be found on Arxiv or on IEEE Xplore.
Browse the Results of the Month archive.