Detection and Tracking of Multipath Channel Parameters Using Belief Propagation
- Published
- Mon, Feb 01, 2021
- Tags
- rotm
- Contact
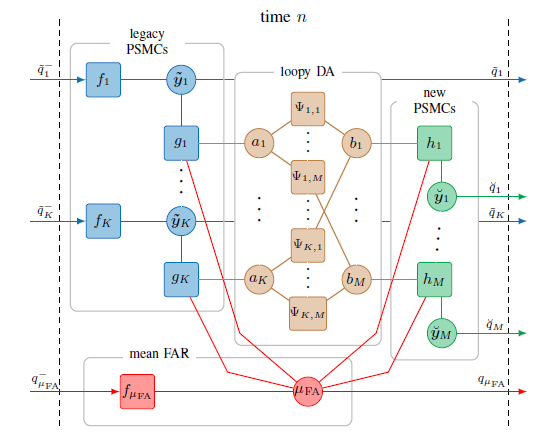
In this work we present a belief propagation (BP) algorithm with probabilistic data association (DA) for detection and tracking of specular multipath components (MPCs). In real dynamic measurement scenarios, the number of MPCs reflected from visible geometric features, the MPC dispersion parameters, and the number of false alarm contributions are unknown and time-varying. We develop a Bayesian model for specular MPC detection and joint estimation problem, and represent it by a factor graph which enables the use of BP for efficient computation of the marginal posterior distributions. A parametric channel estimator is exploited to estimate at each time step a set of MPC parameters, which are further used as noisy measurements by the BP-based algorithm. The algorithm performs probabilistic DA, and joint estimation of the time-varying MPC parameters and mean false alarm rate. Preliminary results using synthetic channel measurements demonstrate the excellent performance of the proposed algorithm in a realistic and very challenging scenario. Furthermore, it is demonstrated that the algorithm is able to cope with a high number of false alarms originating from the prior estimation stage.
Figure: Factor graph representation of the factorized joint posterior pdf shown for time n.
The full version of this paper can be found on Researchgate.
Browse the Results of the Month archive.