Acoustic Scene Classification Using Deep Mixtures Of Pre-trained Convolutional Neural Networks
- Published
- Sat, Feb 01, 2020
- Tags
- rotm
- Contact
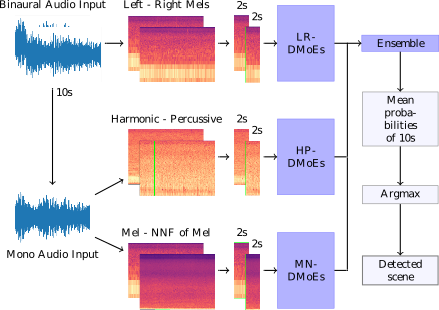
We propose a heterogeneous system of Deep Mixture of Experts (DMoEs) models using different Convolutional Neural Networks (CNNs) for acoustic scene classification (ASC). Each DMoEs module is a mixture of different parallel CNN structures weighted by a gating network. All CNNs use the same input data. The CNN architectures play the role of experts extracting a variety of features. The experts are pre-trained, and kept fixed (frozen) for the DMoEs model. The DMoEs is post-trained by optimizing weights of the gating network, which estimates the contribution of the experts in the mixture. In order to enhance the performance, we use an ensemble of three DMoEs modules each with different pairs of inputs and individual CNN models. The input pairs are spectrogram combinations of binaural audio and mono audio as well as their pre-processed variations using harmonic-percussive source separation (HPSS) and nearest neighbor filters (NNFs). The classification result of the proposed system is 72.1% improving the baseline by around 12% (absolute) on the development data of DCASE 2018 challenge task 1A.
Figure: The system consists of three stages. First, the binaural and mono audio signals are converted to various time-frequency representations chunked into 2s segments. These features are used for training the CNN models and DMoEs. Finally, the probability outputs of these DMoEs models are fed to another ensemble method before making the final label predictions.
Browse the Results of the Month archive.