Frame and Segment Level Recurrent Neural Networks for Phone Classification
- Published
- Fri, Dec 01, 2017
- Tags
- rotm
- Contact
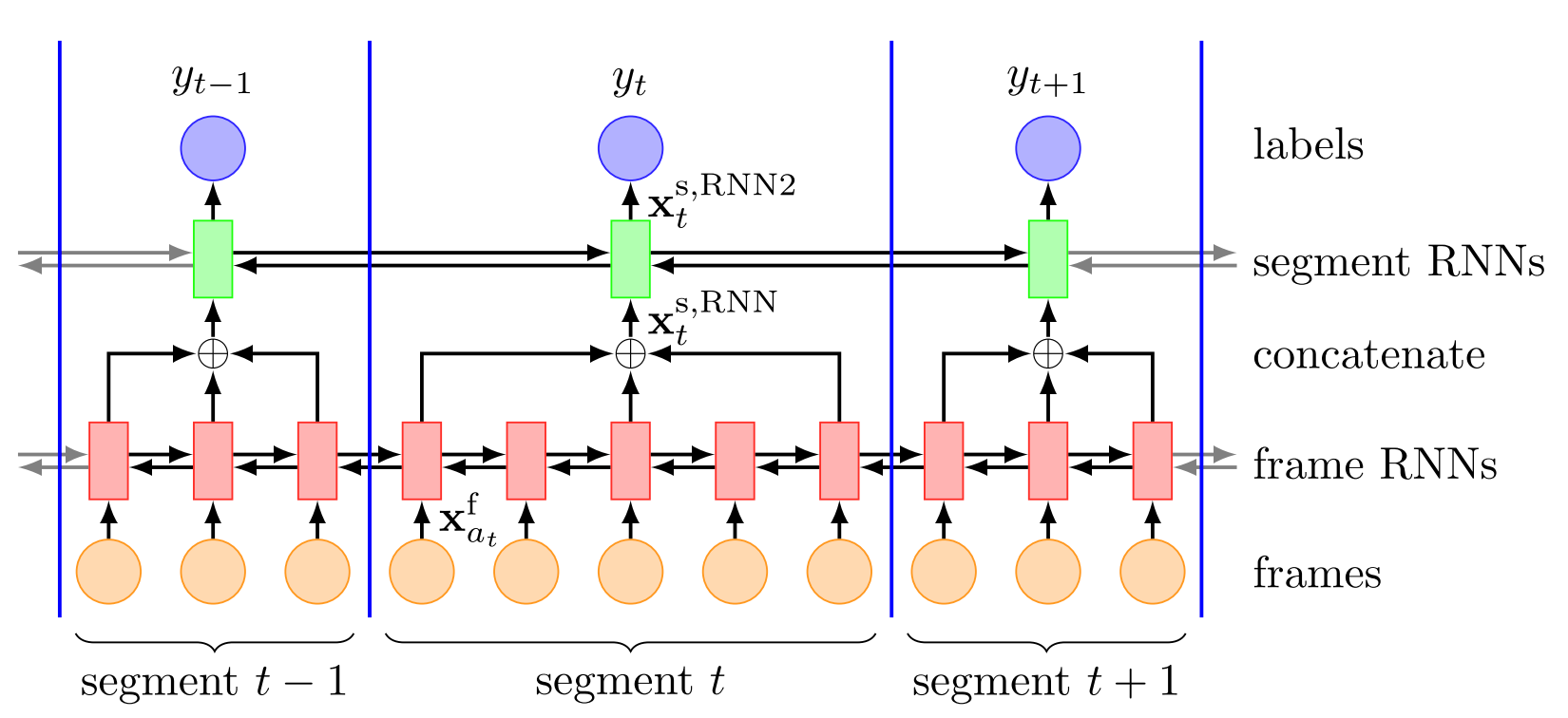
We introduce a simple and efficient frame and segment level RNN model (FS-RNN) for phone classification. It processes the input at frame level and segment level by bidirectional gated RNNs. This type of processing is important to exploit the (temporal) information more effectively compared to (i) models which solely process the input at frame level and (ii) models which process the input on segment level using features obtained by heuristic aggregation of frame level features. Furthermore, we incorporated the activations of the last hidden layer of the FS-RNN as an additional feature type in a neural higher-order CRF (NHO-CRF). In experiments, we demonstrated excellent performance on the TIMIT phone classification task, reporting a performance of 13.8% phone error rate for the FS- RNN model and 11.9% when combined with the NHO-CRF. In both cases we significantly exceeded the state-of-the-art performance.
We gave an oral presentation of this work at Interspeech 2017 in Stockholm. Further, you can download our paper Frame and Segment Level Recurrent Neural Networks for Phone Classification.
Browse the Results of the Month archive.