Structured Regularizer for Neural HigherOrder Sequence Models
- Published
- Sun, Nov 01, 2015
- Tags
- rotm
- Contact
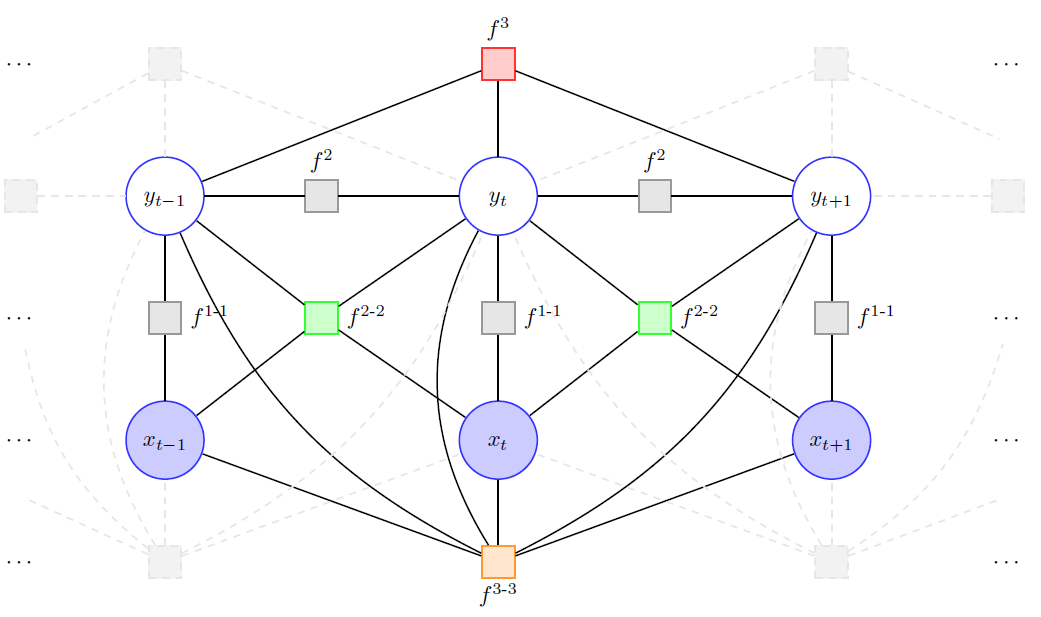
We introduce both joint training of neural higher-order linear-chain conditional random fields (NHO-LC-CRFs) and a new structured regularizer for sequence modelling. We show that this regularizer can be derived as lower bound from a mixture of models sharing parts, e.g. neural sub-networks, and relate it to ensemble learning. Furthermore, it can be expressed explicitly as regularization term in the training objective.
We exemplify its effectiveness by exploring the introduced NHO-LC-CRFs for sequence labeling. Higher-order LC-CRFs with linear factors are well-established for that task, but they lack the ability to model non-linear dependencies. These non-linear dependencies, however, can be efficiently modeled by neural higher-order input-dependent factors. Experimental results for phoneme classification with NHO-LC-CRFs confirm this fact and we achieve state-of-the-art phoneme error rate of 16.7% on TIMIT using the new structured regularizer.
The work has been presented at this year’s ECML – take a look at the full paper [Ratajczak2015b].
Browse the Results of the Month archive.