Neural Higher-Order Factors in Conditional Random Fields for Phoneme Classification
- Published
- Sat, Aug 01, 2015
- Tags
- rotm
- Contact
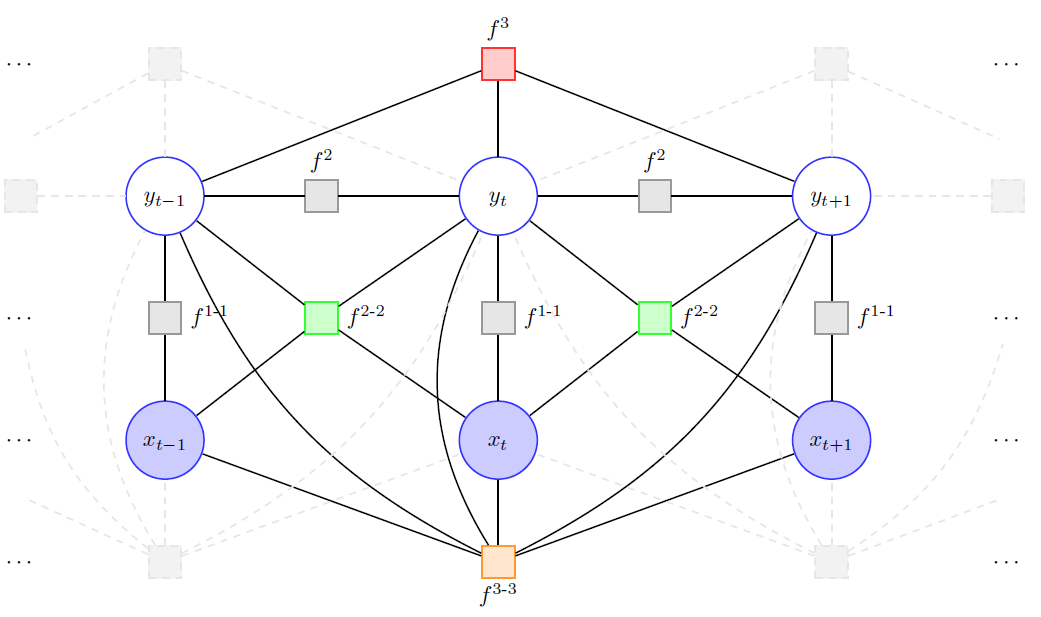
We explore neural higher-order input-dependent factors in linear-chain conditional random fields (LC-CRFs) for sequence labeling, i.e. the fusion of two powerful models. Higher-order LC-CRFs with linear factors are well-established for sequence labeling tasks, but they lack the ability to model non-linear dependencies. These non-linear dependencies, however, can be efficiently modelled by neural higher-order input-dependent factors which map sub-sequences of inputs to sub-sequences of outputs using distinct multilayer perceptron sub-networks. This mapping is important in many tasks, in particular, for phoneme classification where the phone representation strongly depends on the context phonemes. Experimental results for phoneme classification with LC-CRFs and neural higher-order factors confirm this fact and we achieve the best ever reported phoneme classification performance on TIMIT, i.e. a phoneme error rate of 15.8%. Furthermore, we show that the success is not obvious as linear high-order factors degrade phoneme classification performance on TIMIT.
The work has been presented at this year’s Interspeech Conference – take a look at the full paper [Ratajczak2015a].
Browse the Results of the Month archive.