Sum-Product Networks for Structured Prediction: Context-Specific Deep Conditional Random Fields
- Published
- Mon, Sep 01, 2014
- Tags
- rotm
- Contact
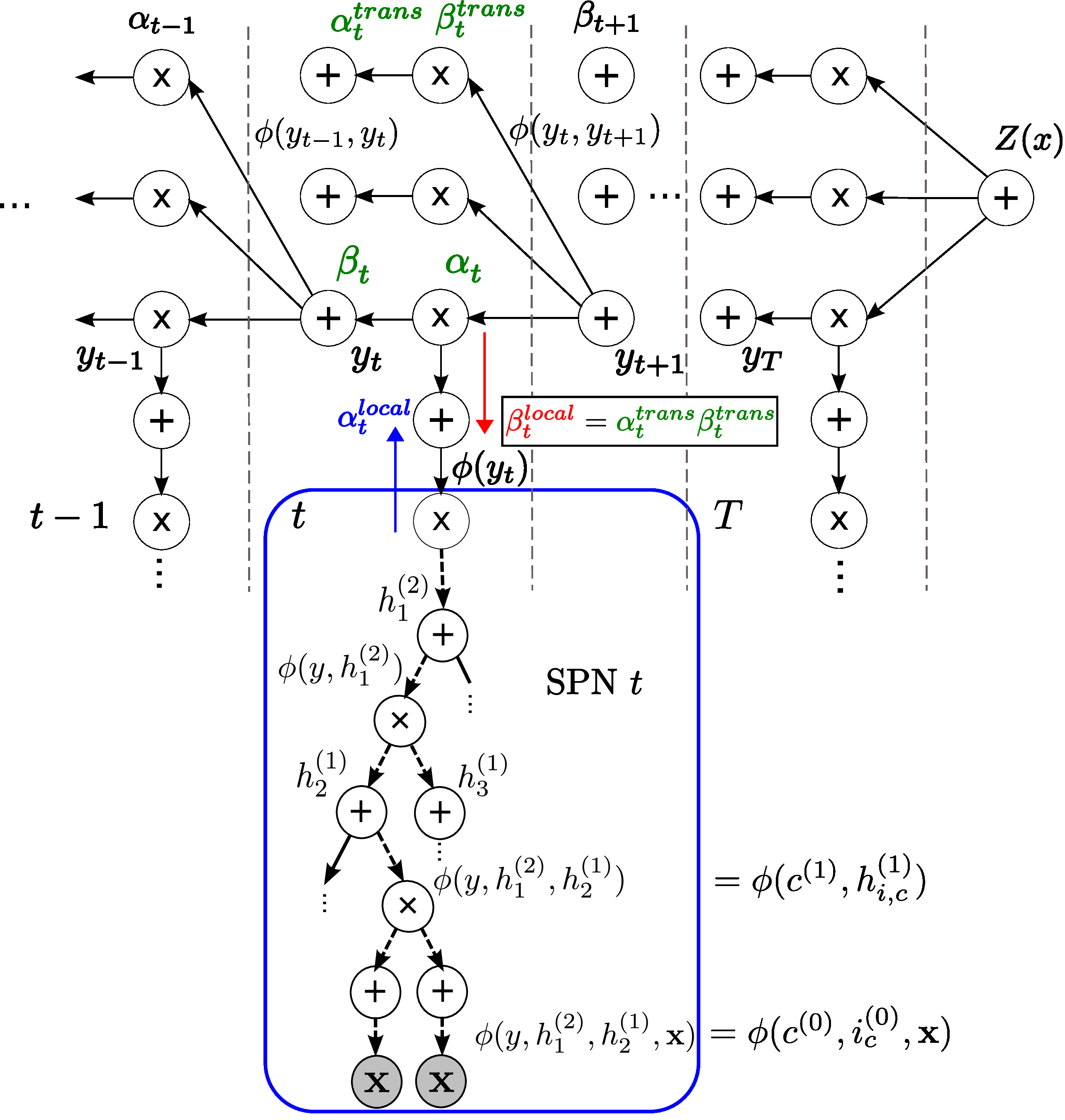
Linear-chain conditional random fields (LC-CRFs) have been successfully applied in many structured prediction tasks. Many previous extensions, e.g. replacing local factors by neural networks, are computationally demanding. In this paper, we extend conventional LC-CRFs by replacing the local factors with sum-product networks, i.e. a promising new deep architecture allowing for exact and efficient inference.
The proposed local factors can be interpreted as an extension of Gaussian mixture models (GMMs). Thus, we provide a powerful alternative to LC-CRFs extended by GMMs. In extensive experiments, we achieved performance competitive to state-of-the-art methods in phone classification and optical character recognition tasks.
The work has been presented at this year’s ICML Workshop (Learning Tractable Probabilistic Models) – take a look at the full paper [Ratajczak2014].
Browse the Results of the Month archive.