Sum Product Networks for Reconstructing Missing Data
- Published
- Tue, Oct 01, 2013
- Tags
- rotm
- Contact
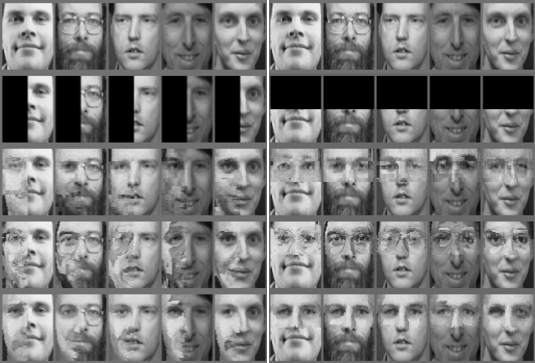
Sum-Product Networks are a novel type of graphical models, which can represent complex variable interaction, still allowing efficient inference. They show especially convincing results in reconstruction tasks, i.e. predicting missing parts of data given partial evidence. The image shows from top to bottom: original image, covered image, reconstruction using Poon & Domingos’ SPN algorithm (2011), Dennis & Ventura’s algorithm (2012), and our recently proposed Greedy Part-wise SPN learning algorithm.
Due to the generative nature of the trained SPNs, they can be applied for versatile inference: the same model can reconstruct the top part, left part, or any other selection of missing variables. For more information, see our recent ECML PKDD paper Greedy Part-Wise Learning of Sum-Product Networks.
Browse the Results of the Month archive.