Information Preserving Markov Chain Aggregation
- Published
- Thu, Aug 01, 2013
- Tags
- rotm
- Contact
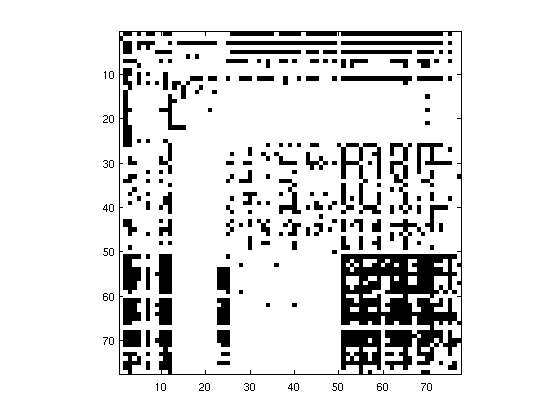
In a recent research collaboration with the Department of Mathematical Structure Theory, we characterized state space aggregations of Markov chains which preserve the information contained in the model. Moreover, we presented an information-theoretic characterization of lumpability, i.e., of the phenomenon that a non-injective function of a Markov chain can be a Markov chain of higher order. These characterizations, together with a set of sufficient conditions on the transition graph of the Markov chain, where employed for lossless model order reduction.
In particular, we trained a letter bi-gram model based on F. Scott Fitzgerald’s text “The great Gatsby” (see the figure for the adjacency matrix of the model; for example, the transitions between lower-case letters on the bottom right can be seen). Applying our algorithm to this model, we identified an aggregation of states which is not only information-preserving, but which renders the output process a second-order Markov chain. With the help of this extremely simple lossless compression method, the entropy of the marginal distribution could be reduced from 4.57 to 4.46 bits. Future work will investigate the performance of our characterization for general n-gram models; in particular, it is of great interest whether a given tri-gram model can be reduced to a tri-gram model on a smaller state space.
This work has been done in collaboration with Christoph Temmel, now at the Department of Mathematics of the VU Amsterdam. For our characterization and our main theoretical results, see “Lumpings of Markov Chains and Entropy Loss” (submitted to J. Appl. Prob.). For the algorithm and the experiments on letter bi-gram models, see “Information-Preserving Markov Aggregations” (accepted for presentation at the IEEE Information Theory Workshop).
Browse the Results of the Month archive.