Sparse Consensus-based Distributed Field Estimation
- Published
- Sat, Oct 01, 2011
- Tags
- rotm
- Contact
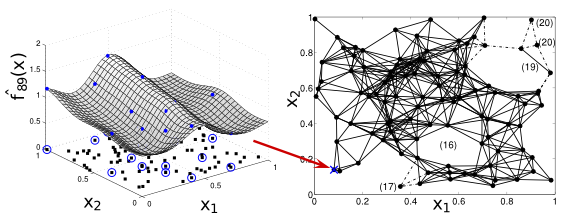
In this work, a fully decentralized algorithm which is inspired by sparse Bayesian learning (SBL) is presented. It can be used for non-parametric sparse estimation of unknown spatial functions -spatial fields- with wireless sensor networks (WSNs). Such a field is represented as a linear combination of weighted fixed basis functions.
The figure shows the estimated field function of one particular sensor. The field function is modeled as a weighted superposition of Gaussian kernels centered at each sensor’s position. The network collaboratively determines which of the kernels are needed, where the centers of the relevant kernels are marked by circles. The solid connection lines depicted on the right represent regions with equivalent sparsity patterns. It can be seen that most of the sensors agree on the same subset of kernels. For more information, please read our paper.
By defining a new probabilistic model for distributed SBL that is built of the connection topology of a WSN, a combination of variational inference and loopy belief propagation is applied to obtain the weights and the sparse subset of relevant basis functions. The algorithm requires only transmission between neighboring sensors and no multi-hop communication is needed. Furthermore, it does not rely on a fixed network structure and no information about the total number of sensors in the network is necessary. Due to consensus in the weight parameters between neighboring nodes, it is demonstrated that also the sparsity patterns of relevant basis functions generally agree across the network.
The paper will be presented at the 5th International Conference on Signal Processing and Communication Systems in December.
Browse the Results of the Month archive.