Lightweight uncertainty modelling using function space particle optimization
- Published
- Thu, Feb 01, 2024
- Tags
- rotm
- Contact
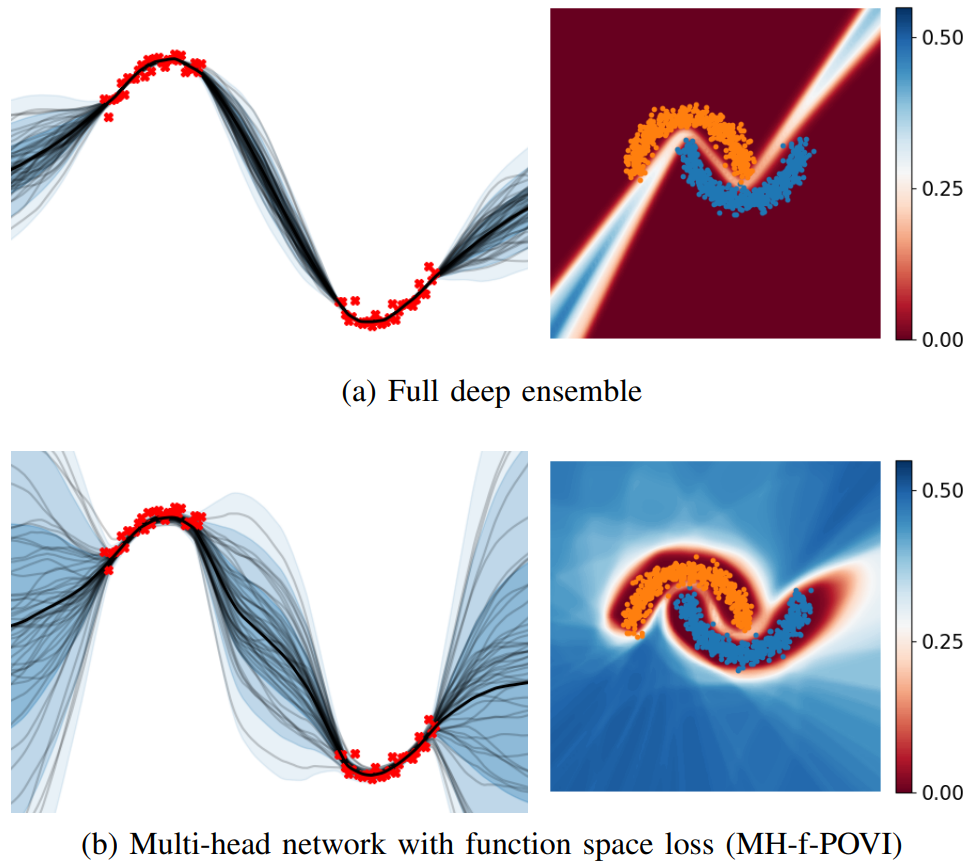
Deep ensembles have shown remarkable empirical success in quantifying uncertainty, albeit at considerable computational cost and memory footprint. Meanwhile, deterministic single-network uncertainty methods have proven as computationally effective alternatives, providing uncertainty estimates based on distributions of latent representations. While those methods are successful at out-of-domain detection, they exhibit poor calibration under distribution shifts. In this work, we propose a method that provides calibrated uncertainty by utilizing particle-based variational inference in function space. Rather than using full deep ensembles to represent particles in function space, we propose a single multi-headed neural network that is regularized to preserve bi-Lipschitz conditions. Sharing a joint latent representation enables a reduction in computational requirements, while prediction diversity is maintained by the multiple heads. We achieve competitive results in disentangling aleatoric and epistemic uncertainty for active learning, detecting out-of-domain data, and providing calibrated uncertainty estimates under distribution shifts while significantly reducing compute and memory requirements.
Browse the Results of the Month archive.